2021년 2월 16일 모바일 게임 매출 순위
Rank | Game | Publisher |
---|---|---|
1 | 리니지M | NCSOFT |
2 | 리니지2M | NCSOFT |
3 | 그랑사가 | NPIXEL |
4 | 세븐나이츠2 | Netmarble |
5 | Cookie Run: Kingdom | Devsisters Corporation |
6 | 기적의 검 | 4399 KOREA |
7 | 라이즈 오브 킹덤즈 | LilithGames |
8 | KartRider Rush+ | NEXON Company |
9 | 뮤 아크엔젤 | Webzen Inc. |
10 | V4 | NEXON Company |
11 | Brawl Stars | Supercell |
12 | 바람의나라: 연 | NEXON Company |
13 | Genshin Impact | miHoYo Limited |
14 | 가디언 테일즈 | Kakao Games Corp. |
15 | Epic Seven | Smilegate Megaport |
16 | 메이플스토리M | NEXON Company |
17 | 미르4 | Wemade Co., Ltd |
18 | R2M | Webzen Inc. |
19 | 블레이드&소울 레볼루션 | Netmarble |
20 | Roblox | Roblox Corporation |
21 | S.O.S:스테이트 오브 서바이벌 | KingsGroup Holdings |
22 | 리니지2 레볼루션 | Netmarble |
23 | FIFA ONLINE 4 M by EA SPORTS™ | NEXON Company |
24 | A3: 스틸얼라이브 | Netmarble |
25 | PUBG MOBILE | KRAFTON, Inc. |
26 | 찐삼국 | ICEBIRD GAMES |
27 | 컴투스프로야구2021 | Com2uS |
28 | 카운터사이드 | NEXON Company |
29 | 검은강호2: 이터널 소울 | 9SplayDeveloper |
30 | 라그나로크 오리진 | GRAVITY Co., Ltd. |
31 | FIFA Mobile | NEXON Company |
32 | AFK 아레나 | LilithGames |
33 | 어비스(ABYSS) | StairGames Inc. |
34 | Pmang Poker : Casino Royal | NEOWIZ corp |
35 | 한게임 포커 | NHN BIGFOOT |
36 | Cookie Run: OvenBreak – Endless Running Platformer | Devsisters Corporation |
37 | 붕괴3rd | miHoYo Limited |
38 | Empires & Puzzles: Epic Match 3 | Small Giant Games |
39 | Gardenscapes | Playrix |
40 | 검은사막 모바일 | PEARL ABYSS |
41 | Age of Z Origins | Camel Games Limited |
42 | 그랑삼국 | YOUZU(SINGAPORE)PTE.LTD. |
43 | Homescapes | Playrix |
44 | 블리치: 만해의 길 | DAMO NETWORK LIMITED |
45 | Lords Mobile: Kingdom Wars | IGG.COM |
46 | 갑부: 장사의 시대 | BLANCOZONE NETWORK KOREA |
47 | Top War: Battle Game | Topwar Studio |
48 | Summoners War | Com2uS |
49 | 랑그릿사 | ZlongGames |
50 | Zombie High School | AWESOMEPIECE |
Loading Multiple Well Log LAS Files Using Python -번역
Python을 사용하여 여러 Well Log LAS 파일로드
Pandas 데이터 프레임에 여러 LAS 파일 추가

Log ASCII Standard (LAS) 파일은 일반적인 Oil & amp;유정 로그 데이터를 저장하고 전송하기위한 가스 산업 형식.내부에 포함 된 데이터는 지하를 분석하고 이해하고 잠재적 인 탄화수소 매장량을 식별하는 데 사용됩니다.이전 기사에서 :웰 로그 데이터로드 및 표시, LASIO 라이브러리를 사용하여 단일 LAS 파일을로드하는 방법을 다루었습니다.
이 기사에서는 다음과 같이 확장합니다. 여러 개의 las 파일을 하위 폴더에서 단일로로드하는 방법을 보여줍니다.팬더 데이터 프레임.이렇게하면 여러 우물의 데이터로 작업하고 matplotlib를 사용하여 데이터를 빠르게 시각화 할 수 있습니다.또한 기계 학습 알고리즘을 실행하기에 적합한 단일 형식으로 데이터를 준비 할 수 있습니다.
이 기사는 내 Python & amp;Petrophysics 시리즈.전체 시리즈의 세부 정보를 찾을 수 있습니다.여기.다음 링크의 GitHub 저장소에서 내 Jupyter 노트북 및 데이터 세트를 찾을 수도 있습니다.
이 기사를 따르기 위해 Jupyter Notebook은 위의 링크에서 찾을 수 있으며이 기사의 데이터 파일은데이터 하위 폴더Python & amp;Petrophysics 저장소.
이 기사에 사용 된 데이터는 공개적으로 액세스 할 수있는네덜란드 NLOG 네덜란드 석유 및 가스 포털.
라이브러리 설정
첫 번째 단계는 우리가 작업 할 도서관을 가져 오는 것입니다.우리는 5 개의 라이브러리를 사용할 것입니다.판다,matplotlib,Seaborn,os, 및Lasio.
Pandas, os 및 lasio는 데이터를로드하고 저장하는 데 사용되는 반면 matplotlib 및 seaborn을 사용하면 우물의 내용을 시각화 할 수 있습니다.
다음으로 모든 las 파일 이름을 저장할 빈 목록을 설정합니다.
둘째,이 예에서는 Data / 15-LASFiles /라는 하위 폴더에 파일이 저장되어 있습니다.이것은 파일이 저장된 위치에 따라 변경됩니다.
이제 우리는os.listdir
메서드를 사용하고 파일 경로를 전달합니다.이 코드를 실행하면 데이터 폴더에있는 모든 파일 목록을 볼 수 있습니다.
이 코드에서 폴더의 내용 목록을 얻습니다.
[ 'L05B03_comp.las',
'L0507_comp.las',
'L0506_comp.las',
'L0509_comp.las',
'WLC_PETRO_COMPUTED_1_INF_1.ASC']
Reading the LAS Files
위에서 볼 수 있듯이 4 개의 LAS 파일과 1 개의 ASC 파일을 반환했습니다.LAS 파일에만 관심이 있으므로 각 파일을 반복하고 확장자가 .las인지 확인해야합니다.또한 확장자가 대문자 인 경우 (.las 대신 .LAS)를 포착하려면 다음을 호출해야합니다..보다 낮은()
파일 확장자 문자열을 소문자로 변환합니다.
파일이 .las로 끝나는지를 확인한 후 파일 이름에 경로 (‘Data / 15-LASFiles /’)를 추가 할 수 있습니다.이는 lasio가 파일을 올바르게 선택하는 데 필요합니다.파일 이름 만 전달하면 독자는 스크립트 또는 노트북과 동일한 디렉토리를보고 결과적으로 실패합니다.
우리가 전화 할 때las_file_list
4 개의 LAS 파일 각각에 대한 전체 경로를 볼 수 있습니다.
[ 'Data / 15-LASFiles / L05B03_comp.las',
'Data / 15-LASFiles / L0507_comp.las',
'Data / 15-LASFiles / L0506_comp.las',
'Data / 15-LASFiles / L0509_comp.las']
Appending Individual LAS Files to a Pandas Dataframe
데이터 프레임에 데이터를 연결 및 / 또는 추가하는 방법에는 여러 가지가 있습니다.이 기사에서는 함께 연결할 데이터 프레임 목록을 만드는 간단한 방법을 사용합니다.
먼저 다음을 사용하여 빈 목록을 만듭니다.df_list = []
.그런 다음 두 번째로 las_file_list를 반복하고 파일을 읽고 데이터 프레임으로 변환합니다.
데이터의 출처를 아는 것이 유용합니다.이 정보를 보관하지 않으면 출처에 대한 정보가없는 데이터로 가득 찬 데이터 프레임이됩니다.이를 위해 새 열을 만들고 웰 이름 값을 할당 할 수 있습니다.lasdf [ 'WELL'] = las.well.WELL.value
.이렇게하면 나중에 데이터를 쉽게 사용할 수 있습니다.
또한 lasio가 데이터 프레임 인덱스를 파일의 깊이 값으로 설정하면 다음과 같은 추가 열을 만들 수 있습니다.깊이
.
이제 목록 객체를 연결하여 LAS 파일의 모든 데이터를 포함하는 작업 데이터 프레임을 만듭니다.
작동중인 데이터 프레임을 호출하면 동일한 데이터 프레임에있는 여러 웰의 데이터가 있음을 알 수 있습니다.

또한 웰 열 내의 고유 한 값을 확인하여 모든 웰이로드되었는지 확인할 수 있습니다.
고유 한 웰 이름의 배열을 반환합니다.
배열 ([ 'L05-B-03', 'L05-07', 'L05-06', 'L05-B-01'], dtype = object)
LAS 파일에 다른 곡선 니모닉이 포함되어있는 경우 (대개 경우) 데이터 프레임에 아직없는 각각의 새 니모닉에 대해 새 열이 생성됩니다.
빠른 데이터 시각화 만들기
이제 데이터를 pandas 데이터 프레임 객체에로드 했으므로 간단하고 빠른 다중 플롯을 만들어 데이터에 대한 통찰력을 얻을 수 있습니다.교차 플롯 / 산점도, 상자 플롯 및 KDE (Kernel Density Estimate) 플롯을 사용하여이를 수행합니다.
이를 시작하려면 먼저 다음을 사용하여 웰 이름별로 데이터 프레임을 그룹화해야합니다.
웰당 교차도 / 산점도
교차 도표 (산점도라고도 함)는 한 변수를 다른 변수에 대해 도표화하는 데 사용됩니다.이 예에서는 중성자 다공성 대 벌크 밀도 교차 플롯을 사용합니다. 이것은 페트로 피학에서 사용되는 매우 일반적인 플롯입니다.
앞서 언급 한 유사한 코드를 사용하여웰 로그 데이터를 사용한 탐색 데이터 분석기사에서는 데이터 프레임의 각 그룹을 반복하고 중성자 다공성 (NPHI) 대 벌크 밀도 (RHOB)의 교차 플롯 (분산도)을 생성 할 수 있습니다.
이렇게하면 4 개의 서브 플롯이있는 다음 이미지가 생성됩니다.
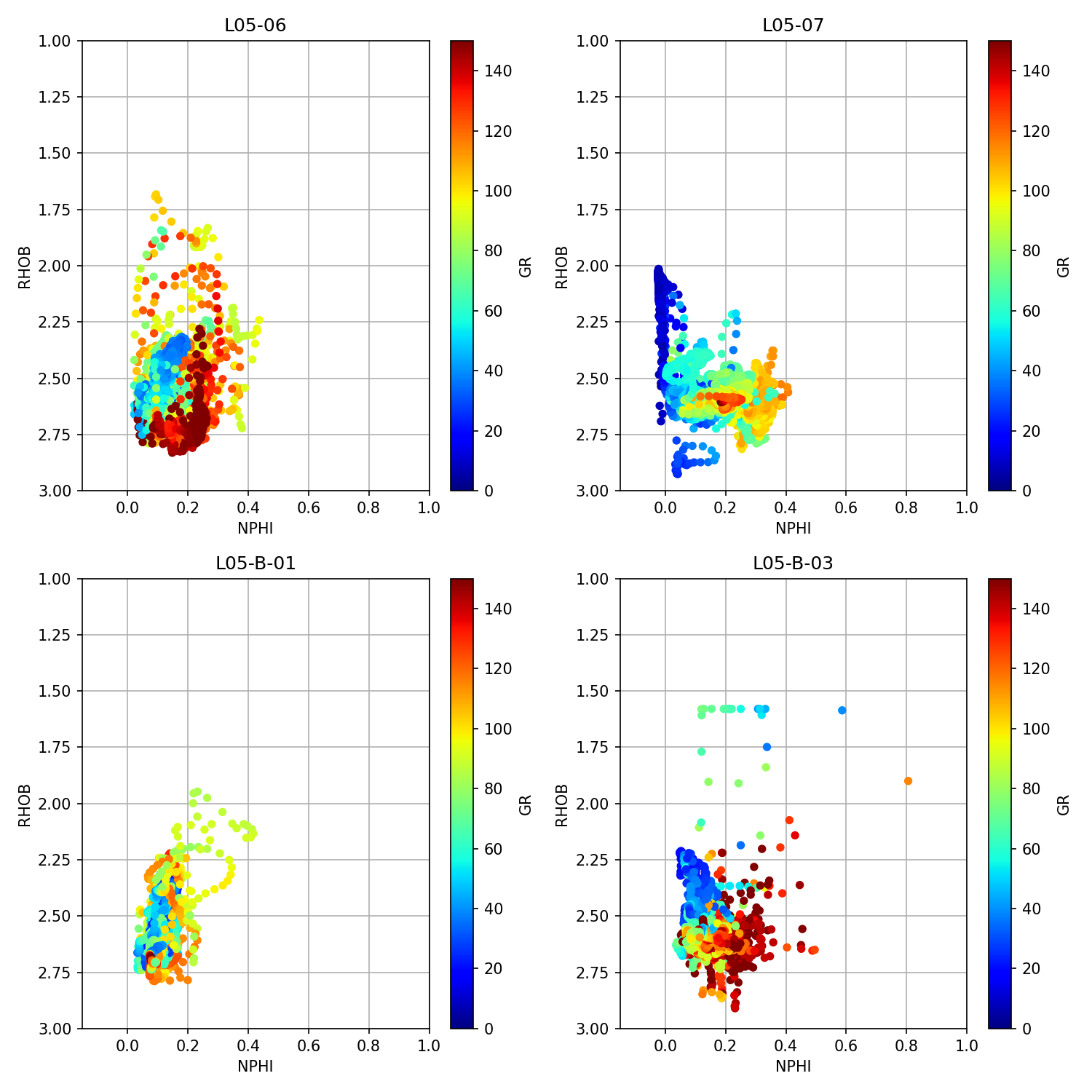
웰당 감마선의 상자 그림
다음으로 모든 우물에서 나온 감마선 큐브의 상자 그림을 표시합니다.박스 플롯은 데이터의 범위 (최소에서 최대까지), 사 분위수 및 데이터의 중앙값을 보여줍니다.
이것은 seaborn 라이브러리에서 한 줄의 코드를 사용하여 달성 할 수 있습니다.인수에서 데이터에 대한 workingdf 데이터 프레임을 전달하고 색조에 대한 WELL 열을 전달할 수 있습니다.후자는 데이터를 각각 고유 한 색상을 가진 개별 상자로 분할합니다.

히스토그램 (커널 밀도 추정치)
마지막으로 히스토그램과 유사한 Kernel Density Estimate 플롯을 사용하여 데이터 프레임에서 곡선 값의 분포를 볼 수 있습니다.
다시 말하지만,이 예제는 groupby 함수를 적용하는 다른 방법을 보여줍니다.x 및 y 제한을 설정하기 위해 matplotlib 함수를 호출하여 플롯을 정리할 수 있습니다.

요약
이 기사에서는 디렉토리에서 .las 확장자를 가진 모든 파일을 검색하여 여러 LAS 파일을로드하고 단일 파일로 연결하는 방법에 대해 설명했습니다.팬더 데이터 프레임.데이터 프레임에이 데이터가 있으면 matplotlib 및 seaborn을 쉽게 호출하여 데이터 시각화를 빠르고 쉽게 이해할 수 있습니다.
읽어 주셔서 감사합니다!
이 기사가 유용하다고 생각되면 Python의 다양한 측면과 로그 데이터를 살펴 보는 다른 기사를 자유롭게 확인하십시오.이 기사 및 기타에서 사용 된 내 코드는GitHub.
연락하고 싶다면 나를 찾을 수 있습니다.LinkedIn 또는 내웹 사이트.
파이썬에 대해 더 많이 배우고 데이터 또는 페트로 피학을 잘 기록하고 싶으십니까?나를 따라와매질.
Loading Multiple Well Log LAS Files Using Python
Loading Multiple Well Log LAS Files Using Python
Appending Multiple LAS Files to a Pandas Dataframe

Log ASCII Standard (LAS) files are a common Oil & Gas industry format for storing and transferring well log data. The data contained within is used to analyze and understand the subsurface, as well as identify potential hydrocarbon reserves. In my previous article: Loading and Displaying Well Log Data, I covered how to load a single LAS file using the LASIO library.
In this article, I expand upon that by showing how to load multiple las files from a subfolder into a single pandas dataframe. Doing this allows us to work with data from multiple wells and visualize the data quickly using matplotlib. It also allows us to prepare the data in a single format that is suitable for running through Machine Learning algorithms.
This article forms part of my Python & Petrophysics series. Details of the full series can be found here. You can also find my Jupyter Notebooks and datasets on my GitHub repository at the following link.
To follow along with this article, the Jupyter Notebook can be found at the link above and the data file for this article can be found in the Data subfolder of the Python & Petrophysics repository.
The data used for this article originates from the publicly accessible Netherlands NLOG Dutch Oil and Gas Portal.
Setting up the Libraries
The first step is to bring in the libraries we will be working with. We will be using five libraries: pandas, matplotlib, seaborn, os, and lasio.
Pandas, os and lasio will be used to load and store our data, whereas matplotlib and seaborn will allow us to visualize the contents of the wells.
Next we are going setup an empty list which will hold all of our las file names.
Secondly, in this example we have our files stored within a sub folder called Data/15-LASFiles/. This will change depending on where your files are stored.
We can now use the os.listdir
method and pass in the file path. When we run this code, we will be able to see a list of all the files in the data folder.
From this code, we get a list of the contents of the folder.
['L05B03_comp.las',
'L0507_comp.las',
'L0506_comp.las',
'L0509_comp.las',
'WLC_PETRO_COMPUTED_1_INF_1.ASC']
Reading the LAS Files
As you can see above, we have returned 4 LAS files and 1 ASC file. As we are only interested in the LAS files we need to loop through each file and check if the extension is .las. Also, to catch any cases where the extension is capitalized (.LAS instead of .las), we need to call upon .lower()
to convert the file extension string to lowercase characters.
Once we have identified if the file ends with .las, we can then add the path (‘Data/15-LASFiles/’) to the file name. This is required for lasio to pick up the files correctly. If we only passed the file name, the reader would be looking in the same directory as the script or notebook, and would fail as a result.
When we call the las_file_list
we can see the full path for each of the 4 LAS files.
['Data/15-LASFiles/L05B03_comp.las',
'Data/15-LASFiles/L0507_comp.las',
'Data/15-LASFiles/L0506_comp.las',
'Data/15-LASFiles/L0509_comp.las']
Appending Individual LAS Files to a Pandas Dataframe
There are a number of different ways to concatenate and / or append data to dataframes. In this article we will use a simple method of create a list of dataframes, which we will concatenate together.
First, we will create an empty list using df_list=[]
. Then secondly, we will loop through the las_file_list, read the file and convert it to a dataframe.
It is useful for us to to know where the data originated. If we didn’t retain this information, we would end up with a dataframe full of data with no information about it’s origins. To do this, we can create a new column and assign the well name value: lasdf['WELL']=las.well.WELL.value
. This will make it easy to work with the data later on.
Additionally, as lasio sets the dataframe index to the depth value from the file, we can create an additional column called DEPTH
.
We will now create a working dataframe containing all of the data from the LAS files by concatenating the list objects.
When we call upon the working dataframe, we can see that we have our data from multiple wells in the same dataframe.

We can also confirm that we have all the wells loaded by checking for the unique values within the well column:
Which returns an array of the unique well names:
array(['L05-B-03', 'L05-07', 'L05-06', 'L05-B-01'], dtype=object)
If our LAS files contain different curve mnemonics, which is often the case, new columns will be created for each new mnemonic that isn’t already in the dataframe.
Creating Quick Data Visualizations
Now that we have our data loaded into a pandas dataframe object, we can create some simple and quick multi-plots to gain insight into our data. We will do this using crossplot/scatterplots, a boxplot and a Kernel Density Estimate (KDE) plot.
To start this, we first need to group our dataframe by the well name using the following:
Crossplot / Scatterplots Per Well
Crossplots (also known as scatterplots) are used to plot one variable against another. For this example we will use a neutron porosity vs bulk density crossplot, which is a very common plot used in petrophysics.
Using a similar piece of code that was previously mentioned on my Exploratory Data Analysis With Well Log Data article, we can loop through each of the groups in the dataframe and generate a crossplot (scatter plot) of neutron porosity (NPHI) vs bulk density (RHOB).
This generates the following image with 4 subplots:
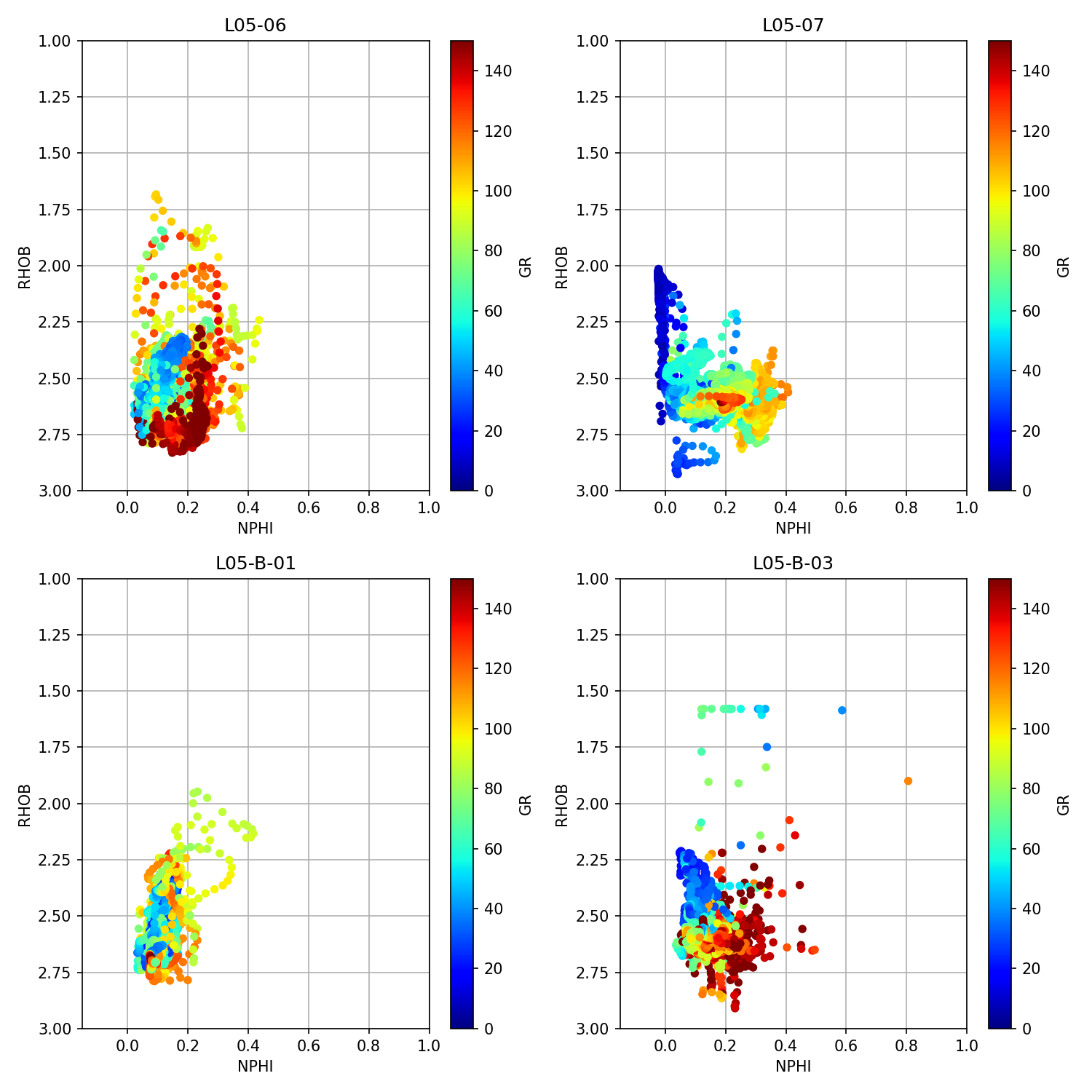
Boxplot of Gamma Ray Per Well
Next up, we will display a boxplot of the gamma ray cuvre from all wells. The box plot will show us the extent of the data (minimum to maximum), the quartiles, and the median value of the data.
This can be achieved using a single line of code in the seaborn library. In the arguments we can pass in the workingdf dataframe for data, and the WELL column for the hue. The latter of which will split the data up into individual boxes, each with it’s own unique color.

Histogram (Kernel Density Estimate)
Finally, we can view the distribution of the values of a curve in the dataframe by using a Kernel Density Estimate plot, which is similar to a histogram.
Again, this example shows another way to apply the groupby function. We can tidy up the plot by calling up matplotlib functions to set the x and y limits.

Summary
In this article we have covered how to load multiple LAS files by searching a directory for all files with a .las extension and concatenate them into a single pandas dataframe. Once we have this data in a dataframe, we can easily call upon matplotlib and seaborn to make quick and easy to understand visualizations of the data.
Thanks for reading!
If you have found this article useful, please feel free to check out my other articles looking at various aspects of Python and well log data. You can also find my code used in this article and others at GitHub.
If you want to get in touch you can find me on LinkedIn or at my website.
Interested in learning more about python and well log data or petrophysics? Follow me on Medium.
2021년 2월 15일 모바일 게임 매출 순위
Rank | Game | Publisher |
---|---|---|
1 | 리니지M | NCSOFT |
2 | 리니지2M | NCSOFT |
3 | 그랑사가 | NPIXEL |
4 | 세븐나이츠2 | Netmarble |
5 | Cookie Run: Kingdom | Devsisters Corporation |
6 | 기적의 검 | 4399 KOREA |
7 | Genshin Impact | miHoYo Limited |
8 | R2M | Webzen Inc. |
9 | 라이즈 오브 킹덤즈 | LilithGames |
10 | V4 | NEXON Company |
11 | 바람의나라: 연 | NEXON Company |
12 | 메이플스토리M | NEXON Company |
13 | 뮤 아크엔젤 | Webzen Inc. |
14 | KartRider Rush+ | NEXON Company |
15 | S.O.S:스테이트 오브 서바이벌 | KingsGroup Holdings |
16 | 미르4 | Wemade Co., Ltd |
17 | 리니지2 레볼루션 | Netmarble |
18 | 블레이드&소울 레볼루션 | Netmarble |
19 | 어비스(ABYSS) | StairGames Inc. |
20 | Pmang Poker : Casino Royal | NEOWIZ corp |
21 | FIFA ONLINE 4 M by EA SPORTS™ | NEXON Company |
22 | 찐삼국 | ICEBIRD GAMES |
23 | Roblox | Roblox Corporation |
24 | 컴투스프로야구2021 | Com2uS |
25 | PUBG MOBILE | KRAFTON, Inc. |
26 | Brawl Stars | Supercell |
27 | 카운터사이드 | NEXON Company |
28 | 한게임 포커 | NHN BIGFOOT |
29 | 검은강호2: 이터널 소울 | 9SplayDeveloper |
30 | A3: 스틸얼라이브 | Netmarble |
31 | Cookie Run: OvenBreak – Endless Running Platformer | Devsisters Corporation |
32 | AFK 아레나 | LilithGames |
33 | 그랑삼국 | YOUZU(SINGAPORE)PTE.LTD. |
34 | Lords Mobile: Kingdom Wars | IGG.COM |
35 | Age of Z Origins | Camel Games Limited |
36 | 블리치: 만해의 길 | DAMO NETWORK LIMITED |
37 | Gardenscapes | Playrix |
38 | 라그나로크 오리진 | GRAVITY Co., Ltd. |
39 | Dungeon Knight: 3D Idle RPG | mobirix |
40 | Homescapes | Playrix |
41 | Random Dice: PvP Defense | 111% |
42 | Empires & Puzzles: Epic Match 3 | Small Giant Games |
43 | Top War: Battle Game | Topwar Studio |
44 | Summoners War | Com2uS |
45 | 한게임포커 클래식 with PC | NHN Corp. |
46 | 명일방주 | Yostar Limited. |
47 | 검은사막 모바일 | PEARL ABYSS |
48 | 갑부: 장사의 시대 | BLANCOZONE NETWORK KOREA |
49 | FIFA Mobile | NEXON Company |
50 | 모두의마블 | Netmarble |