2021년 2월 18일 모바일 게임 매출 순위
Rank | Game | Publisher |
---|---|---|
1 | 리니지M | NCSOFT |
2 | 리니지2M | NCSOFT |
3 | 그랑사가 | NPIXEL |
4 | 기적의 검 | 4399 KOREA |
5 | 세븐나이츠2 | Netmarble |
6 | Cookie Run: Kingdom | Devsisters Corporation |
7 | 라이즈 오브 킹덤즈 | LilithGames |
8 | 뮤 아크엔젤 | Webzen Inc. |
9 | KartRider Rush+ | NEXON Company |
10 | Brawl Stars | Supercell |
11 | Genshin Impact | miHoYo Limited |
12 | 바람의나라: 연 | NEXON Company |
13 | V4 | NEXON Company |
14 | 가디언 테일즈 | Kakao Games Corp. |
15 | Epic Seven | Smilegate Megaport |
16 | Roblox | Roblox Corporation |
17 | 미르4 | Wemade Co., Ltd |
18 | 메이플스토리M | NEXON Company |
19 | 블레이드&소울 레볼루션 | Netmarble |
20 | A3: 스틸얼라이브 | Netmarble |
21 | 리니지2 레볼루션 | Netmarble |
22 | R2M | Webzen Inc. |
23 | FIFA ONLINE 4 M by EA SPORTS™ | NEXON Company |
24 | S.O.S:스테이트 오브 서바이벌 | KingsGroup Holdings |
25 | PUBG MOBILE | KRAFTON, Inc. |
26 | 찐삼국 | ICEBIRD GAMES |
27 | 라그나로크 오리진 | GRAVITY Co., Ltd. |
28 | 카운터사이드 | NEXON Company |
29 | 검은강호2: 이터널 소울 | 9SplayDeveloper |
30 | Cookie Run: OvenBreak – Endless Running Platformer | Devsisters Corporation |
31 | FIFA Mobile | NEXON Company |
32 | Empires & Puzzles: Epic Match 3 | Small Giant Games |
33 | 붕괴3rd | miHoYo Limited |
34 | 컴투스프로야구2021 | Com2uS |
35 | 그랑삼국 | YOUZU(SINGAPORE)PTE.LTD. |
36 | AFK 아레나 | LilithGames |
37 | Homescapes | Playrix |
38 | 어비스(ABYSS) | StairGames Inc. |
39 | Pmang Poker : Casino Royal | NEOWIZ corp |
40 | 한게임 포커 | NHN BIGFOOT |
41 | Gardenscapes | Playrix |
42 | Age of Z Origins | Camel Games Limited |
43 | 검은사막 모바일 | PEARL ABYSS |
44 | 블리치: 만해의 길 | DAMO NETWORK LIMITED |
45 | 갑부: 장사의 시대 | BLANCOZONE NETWORK KOREA |
46 | Zombie High School | AWESOMEPIECE |
47 | Top War: Battle Game | Topwar Studio |
48 | 프린세스 커넥트! Re:Dive | Kakao Games Corp. |
49 | 달빛조각사 | Kakao Games Corp. |
50 | Lords Mobile: Kingdom Wars | IGG.COM |
How Do Data Scientists Use Twitter? Let Us Count the Ways -번역
읽기 목록
데이터 과학자들은 Twitter를 어떻게 사용합니까?방법을 세어 보자
TDS 아카이브에서 최고의 Twitter 데이터 분석을 찾아보세요.

일화로, 트위터에있을 수 있거나 삶에서 기쁨, 건강 및 균형을 찾으려고 시도 할 수 있지만 둘다는 아닙니다.내 타임 라인의 작가, 언론인, 틈새 음식에 대한 의견이 많은 사람들은 상당히 다양한 라인업을 형성합니다.우리를 하나로 묶는 것은 공간 그 자체에 대한 강한 양면성입니다. 예, 여기서 말을하겠습니다. 우리가 잊을 수없는“지옥”입니다.
과거의 TDS 아카이브 파헤 치기에프몇 주 동안 다른 가능성, 심지어 대체 현실을 제시하는 예상치 못한 효과가있었습니다.여기 수십 명의 데이터 과학자와 AI 전문가가 트위터에서 엄청난 시간을 보내고 … 그것으로 생산적인 일을하고 있습니까?!아니절망의 나선에 빠지는 것?!세상에 대한 사려 깊은 통찰력 그리기?!?!
그게 어떻게 가능 했습니까?
뉴스의 주요 소스로 플랫폼을 사용함으로써 제 인식이 크게 달라 졌다는 것이 이제 분명합니다.당신이 주로 트위터에 가면 [무작위로 무차별 적으로 손을 흔들어 세상에], 뉴스에 대한 감정이 결국 앱에 대해 느끼는 감정과 수렴하는 이유가 될 것입니다.반대로 한 걸음 물러서서다른사람과 커뮤니티는 플랫폼을 사용합니다.트위터는 강력한 API 덕분에 가능했습니다.-저도 언젠가 달성하기를 희망하는 행복한 분리 (또는 적어도 그것의 유사성)에 자신을 부여합니다.
내 요점을 증명하기 위해 내가 가장 좋아하는 TDS Twitter / 데이터 과학 크로스 오버 게시물이 있습니다. 읽어보세요!그들 모두, 그들 중 일부, 커플;후회하지 않을 것입니다.아카이브 실행매우깊은, 그래서 저는 몇 가지 주요 필터링을 거쳐 새롭고 주목할만한 실습 리소스 및 역대 최고의 제품이라는 세 가지 범주에 걸쳐이 컬렉션을 모았습니다.뛰어 들자.
새롭고 주목할만한
내가 주로 뉴스 소비에 트위터를 사용한다고 언급 했나요?그 이유는 정치적, 문화적 순간을 포착하는 플랫폼의 부인할 수없는 능력 때문입니다.이다순간.Black Lives Matter (그리고 때때로 기업이 사회 운동에 참여하는 방식)부터 트위터 사용자의 넷플릭스 히트작에 대한 환호 한 반응까지Bridgerton휴일 동안이 최근 게시물은 데이터 과학 도구를 적용하여 그러한 순간을 깊이 연구하고 매우 잘 수행합니다.
실습 리소스
많은 독자들이 TDS를 찾는 이유는 우리 커뮤니티가 자신의 업무, 연구 또는 열정 프로젝트에서 직면 한 실질적인 도전에 대한 답을 찾는 곳이기 때문입니다.트위터 데이터 분석도 예외는 아니며 여기에 수집 된 게시물은 트윗에서 통찰력을 수집, 정리, 처리 및 도출하는 방법에 대한 명확성과 단계별 지침을 제공합니다.
12 가지 트위터 감정 분석 알고리즘 비교
트윗 분류의 정확성에 대해 12 가지 감정 분석 알고리즘을 비교했습니다.fasText 딥 러닝…
towardsdatascience.com
Twitter Ego-Networks & amp;자아 커뮤니티 감지
Twitter 네트워크에서 커뮤니티 감지에 대한 그래프 기반 접근 방식
towardsdatascience.com
역대 최고의 인물
예,이 주제에 대한 우리의 아카이브는 방대하지만 대부분의 트윗과 달리 일부 게시물은 실제로 시간의 시험을 견뎌냈으며 작성자가 처음 게시했을 때와 마찬가지로 오늘날에도 날카 롭고 매력적입니다.트위터의 자체 채용 프로세스부터 트윗의 언어 적 표시에서 우울증 징후 감지에 이르기까지 다양한 주제를 다룹니다.하지만 먼저 :귀여운 강아지.
특히 위의 사항 중 하나가 귀하에게 반향을 불러 일으켰다면 알려주십시오.또한 : 여기 또는 다른 사이트에서 Twitter 관련 데이터 프로젝트를 다루는 훌륭한 게시물을 읽었습니까?직접 작성 했습니까?의견에서 우리와 공유하십시오.또한-향후 읽기 목록에서 다루고 싶은 다른 주제가 있습니까?그것도 말 해주세요.
How Do Data Scientists Use Twitter? Let Us Count the Ways
Reading List
How Do Data Scientists Use Twitter? Let Us Count the Ways
Discover some of the best Twitter data analyses from the TDS archive.

Anecdotally, it would seem that you can be on Twitter, or you can attempt to find joy, health, and balance in your life, but not both. The writers, journalists, and niche food-opinion-havers in my timeline form a fairly diverse lineup. What unites us is a strong ambivalence—yes, I’m mincing words here—about the space itself: the “hellsite” we can’t leave behind.
Digging into the TDS archive these past few weeks has had the unexpected effect of suggesting a different possibility—an alternate reality, even. Here were dozens of data scientists and AI experts spending massive amounts of time on Twitter and… doing productive things with it?! Not falling into ever-wilder spirals of despair?! Drawing thoughtful insights about the world?!?!
How was any of that possible?
It’s clear to me now that my use of the platform as a primary source of news has dramatically shaped my perception of it. If you mostly go on to Twitter to stay abreast of [waves hands randomly and indiscriminately at the world], it would stand to reason that the emotions you have about the news eventually converge with the ones you feel about the app. On the flip side, taking a step back to analyze how other people and communities use the platform — which Twitter has made possible thanks to its robust API—lends itself to blissful detachment (or at least a semblance of it), one that I one day hope to achieve as well.
To prove my point, here are some of my favorite TDS Twitter/data science crossover posts — read them! All of them, some of them, a couple; you won’t regret it. The archive runs extremely deep, so I assembled this collection after some major filtering, and across three categories: new and noteworthy, hands-on resources, and all-time greats. Let’s dive in.
New and noteworthy
Did I mention I use Twitter mostly for news consumption? The reason is the platform’s undeniable ability to capture political and cultural moments — sometimes it is the moment. From Black Lives Matter (and the occasionally fraught ways companies have attached themselves to the social movement), to Twitter users’ jubilant reception of Netflix hit Bridgerton over the holidays, these recent posts apply the tools of data science to study such moments in depth, and do it extremely well.
Hands-on resources
Many of our readers come to TDS because our community is where they find answers to the practical challenges they face in their work, in their studies, or in their passion projects. Twitter data analysis is no exception, and the posts gathered here provide clarity and step-by-step instructions on how to collect, clean, process, and draw insights from tweets.
All-time greats
Yes, our archive on this topic is immense, but some posts — unlike most tweets!—have really stood the test of time, and are just as sharp and engaging today as they were when their authors first published them. They cover a wide range of topics, from Twitter’s own hiring process to detecting signs of depression in tweets’ linguistic markers. But first: CUTE DOGS.
Let us know if any of the above has resonated with you in particular. Also: have you read a great post—here or on another site—featuring a Twitter-related data project? Have you written one yourself? Share it with us in the comments. Also-also: are there other topics you’d like to see us cover in a future reading list? Tell us that, too.
2021년 2월 18일 성남시 분당구 부동산 경매 데이터 분석
경기도 성남시 분당구 분당로 190, 105동 3층308호 (분당동,샛별마을) [집합건물 철근콘크리트조 58.17㎡]
항목 | 값 |
---|---|
경매번호 | 2020타경7028 |
경매날짜 | 2021.03.02 |
법원 | 성남지원 |
담당 | 경매7계 |
감정평가금액 | 665,000,000 |
경매가 | 665,000,000(100%) |
유찰여부 | 신건 |
<최근 1년 실거래가 정보>
– 총 거래 수: 50건
– 동일 평수 거래 수: 11건
최근 1년 동일 평수 거래건 보기
날짜 | 전용면적 | 층 | 가격 |
---|---|---|---|
2020-10-07 | 58.17 | 5 | 71000 |
2020-08-10 | 58.17 | 6 | 72000 |
2020-08-03 | 58.17 | 4 | 60000 |
2020-07-06 | 58.17 | 3 | 64500 |
2020-06-18 | 58.17 | 15 | 63500 |
2020-06-02 | 58.17 | 2 | 59500 |
2020-06-05 | 58.17 | 4 | 60000 |
2020-05-16 | 58.17 | 9 | 60500 |
2020-03-10 | 58.17 | 12 | 62800 |
2020-02-01 | 58.17 | 11 | 57900 |
2020-02-18 | 58.17 | 12 | 59500 |
경기도 성남시 분당구 서판교로 29, 914동 8층802호 (판교동,판교원마을) [집합건물 철근콘크리트구조 84.9505 ㎡]
항목 | 값 |
---|---|
경매번호 | 2020타경4005 |
경매날짜 | 2021.03.02 |
법원 | 성남지원 |
담당 | 경매7계 |
감정평가금액 | 1,110,000,000 |
경매가 | 1,110,000,000(100%) |
유찰여부 | 신건 |
<최근 1년 실거래가 정보>
– 총 거래 수: 58건
– 동일 평수 거래 수: 35건
최근 1년 동일 평수 거래건 보기
날짜 | 전용면적 | 층 | 가격 |
---|---|---|---|
2021-01-22 | 84.9562 | 12 | 150000 |
2021-01-23 | 84.9958 | 10 | 150000 |
2020-12-29 | 84.9562 | 10 | 150000 |
2020-12-04 | 84.9958 | 15 | 147500 |
2020-12-07 | 84.9505 | 10 | 148500 |
2020-11-06 | 84.9562 | 5 | 135000 |
2020-10-13 | 84.9505 | 11 | 120000 |
2020-10-17 | 84.9562 | 1 | 120000 |
2020-10-25 | 84.9958 | 19 | 138000 |
2020-10-26 | 84.9505 | 16 | 136500 |
2020-10-31 | 84.9958 | 18 | 145000 |
2020-09-01 | 84.9505 | 1 | 114500 |
2020-09-05 | 84.9907 | 15 | 128000 |
2020-08-21 | 84.9907 | 14 | 129000 |
2020-08-26 | 84.9907 | 10 | 131500 |
2020-08-27 | 84.9562 | 10 | 133500 |
2020-07-16 | 84.9907 | 9 | 124500 |
2020-07-25 | 84.9562 | 13 | 119250 |
2020-07-26 | 84.9562 | 12 | 125000 |
2020-07-03 | 84.9958 | 12 | 116000 |
2020-07-04 | 84.9907 | 13 | 117000 |
2020-07-07 | 84.9505 | 5 | 120000 |
2020-06-12 | 84.9505 | 11 | 114000 |
2020-06-13 | 84.9958 | 9 | 109700 |
2020-06-17 | 84.9907 | 10 | 120000 |
2020-06-17 | 84.9907 | 12 | 110000 |
2020-06-20 | 84.9907 | 15 | 114000 |
2020-06-22 | 84.9958 | 7 | 107000 |
2020-06-30 | 84.9907 | 4 | 113000 |
2020-06-05 | 84.9505 | 8 | 115000 |
2020-06-06 | 84.9505 | 2 | 106000 |
2020-06-09 | 84.9907 | 5 | 109000 |
2020-03-14 | 84.9907 | 1 | 101000 |
2020-03-27 | 84.9907 | 16 | 100200 |
2020-02-14 | 84.9958 | 8 | 113000 |
2021년 2월 17일 모바일 게임 매출 순위
Rank | Game | Publisher |
---|---|---|
1 | 리니지M | NCSOFT |
2 | 리니지2M | NCSOFT |
3 | 그랑사가 | NPIXEL |
4 | 세븐나이츠2 | Netmarble |
5 | Cookie Run: Kingdom | Devsisters Corporation |
6 | 기적의 검 | 4399 KOREA |
7 | 라이즈 오브 킹덤즈 | LilithGames |
8 | KartRider Rush+ | NEXON Company |
9 | 뮤 아크엔젤 | Webzen Inc. |
10 | V4 | NEXON Company |
11 | Brawl Stars | Supercell |
12 | 바람의나라: 연 | NEXON Company |
13 | Genshin Impact | miHoYo Limited |
14 | 가디언 테일즈 | Kakao Games Corp. |
15 | Epic Seven | Smilegate Megaport |
16 | 메이플스토리M | NEXON Company |
17 | 미르4 | Wemade Co., Ltd |
18 | R2M | Webzen Inc. |
19 | 블레이드&소울 레볼루션 | Netmarble |
20 | Roblox | Roblox Corporation |
21 | S.O.S:스테이트 오브 서바이벌 | KingsGroup Holdings |
22 | 리니지2 레볼루션 | Netmarble |
23 | FIFA ONLINE 4 M by EA SPORTS™ | NEXON Company |
24 | A3: 스틸얼라이브 | Netmarble |
25 | PUBG MOBILE | KRAFTON, Inc. |
26 | 찐삼국 | ICEBIRD GAMES |
27 | 컴투스프로야구2021 | Com2uS |
28 | 카운터사이드 | NEXON Company |
29 | 검은강호2: 이터널 소울 | 9SplayDeveloper |
30 | 라그나로크 오리진 | GRAVITY Co., Ltd. |
31 | FIFA Mobile | NEXON Company |
32 | AFK 아레나 | LilithGames |
33 | 어비스(ABYSS) | StairGames Inc. |
34 | Pmang Poker : Casino Royal | NEOWIZ corp |
35 | 한게임 포커 | NHN BIGFOOT |
36 | Cookie Run: OvenBreak – Endless Running Platformer | Devsisters Corporation |
37 | 붕괴3rd | miHoYo Limited |
38 | Empires & Puzzles: Epic Match 3 | Small Giant Games |
39 | Gardenscapes | Playrix |
40 | 검은사막 모바일 | PEARL ABYSS |
41 | Age of Z Origins | Camel Games Limited |
42 | 그랑삼국 | YOUZU(SINGAPORE)PTE.LTD. |
43 | Homescapes | Playrix |
44 | 블리치: 만해의 길 | DAMO NETWORK LIMITED |
45 | Lords Mobile: Kingdom Wars | IGG.COM |
46 | 갑부: 장사의 시대 | BLANCOZONE NETWORK KOREA |
47 | Top War: Battle Game | Topwar Studio |
48 | Summoners War | Com2uS |
49 | 랑그릿사 | ZlongGames |
50 | Zombie High School | AWESOMEPIECE |
Georgia Tech’s MS Analytics Program: My Review Part II -번역
Georgia Tech의 MS Analytics 프로그램 : My Review Part II

첫 글을 쓴 후리뷰OMSA 프로그램의 많은 예비 학생들과 현재 학생들과 연락을 받았습니다.이 프로그램은 다양한 배경을 가진 학생들을 끌어 들이기 때문에 모든 사람에게 적용되는 조언을 제공하는 것은 어려울 수 있습니다.다음은 제가받는 가장 일반적인 질문과 그에 대한 답변입니다.
OMSA가 나에게 적합한 프로그램입니까?
프로그램 설명을 읽으면디동의 개요페이지, 그들은 “학제 간”이라는 설명자를 사용합니다.저는 이것이 프로그램에 대한 완벽한 단어 선택이라고 생각합니다.이 프로그램에서 다루는 주제의 범위는 다양한 분야에서 나옵니다.그만큼필수과정은 재무, 회계, 객체 지향 프로그래밍, 데이터 분석, 기계 학습, 통계, 웹 개발, 클라우드 컴퓨팅, 데이터 정리, 스크립팅 언어 및 데이터 시각화를 다룹니다.이 고도로 기술적 인 프로그램의 강조점은 깊이에 대한 폭입니다.그게 매력적으로 들리면 이것이 당신을위한 프로그램 일 수 있습니다.그러나 전문가가되고 이러한 개별 분야 중 하나를 전문으로하고 싶다면이 프로그램보다 더 나은 옵션이 있습니다.
이 프로그램은 폭을 강조하지만 자료의 난이도를 과소 평가하지 마십시오.비즈니스, 컴퓨터 과학 및 통계 분야에서 유능하다는 것은 쉬운 일이 아닙니다.종종 개인은이 프로그램에서 다루는 분야 중 하나 이상으로 어려움을 겪습니다.학위의 기술적 엄격함에 관심이 있다면 등록 전에 기술을 향상 시키거나 다른 프로그램을 고려할 것을 권합니다.다른 온라인 정량 석사 학위에는 데이터 과학, 응용 경제학, 통계, 컴퓨터 과학, 비즈니스 분석 및 MBA : 분석 집중이 포함됩니다.대학의 비즈니스 스쿨에서 실행되는 분석 프로그램은 수학 및 컴퓨터 과학에서 덜 엄격 할 것입니다.
어떤 OMSA 전문화를 선택해야합니까?
필수 과정을 마치면 3 가지 전문화 중에서 선택할 수 있습니다.전문화라는 단어는 대부분의 프로그램이 모든 학생에게 동일하기 때문에 약간 오해의 소지가 있습니다.해당 전문 분야에는 6/36 크레딧 만 제공됩니다.그렇지 않으면 나머지는 필요하거나 귀하의 재량에 달려 있습니다.다음과 같이 세 가지 전문 분야를 빠르게 요약 할 수 있습니다.

난이도가 다소 주관적이지만 대부분의 학생들이 내 평가에 동의 할 것이라고 생각합니다.컴퓨터 과학 과정은 주당 가장 많은 시간을 요구하는 경향이 있습니다.
추천하고 싶은 특별한 수업 순서가 있습니까?
이 프로그램에서 다루는 다양한 분야가 너무 많기 때문에 적절한 수업 순서를 결정하기가 까다로울 수 있습니다.결정하는 동안 고려해야 할 많은 변수가 있습니다.다음은 이상적인 2 ~ 4 년 대학원 계획을 세우기 전에 답해야 할 몇 가지 질문입니다.
한 학기에 몇 개의 수업을들을 수 있습니까?
평균 작업량은 코스에 따라 3 학점당 주당 8-20 시간입니다.수업 기대치에 따라 어떤 수업을 함께 사용할 수 있는지 고려해야합니다.귀하의 개인 시간 약속은 해당 과목의 기술 강도에 따라 평균과 다릅니다.예를 들어 통계에 대한 배경 지식이 풍부하다면 통계 과정에 평균 이하의 시간을 할애 할 수 있습니다.
매 학기에 어떤 수업이 제공됩니까?
여름 학기는 가을과 봄보다 짧습니다.따라서 전체 수업 목록의 하위 집합 인 더 빠른 속도로 이동할 수있는 과정 만 제공합니다.더 빠른 속도로 실행되기 때문에 주당 평균 워크로드는 평균보다 약 20 % 높습니다.
가까이에서 수강하기에 가장 적합한 수업은 무엇입니까?
서로 밀접하게 관련된 두 가지 분야를 취하는 것이 훨씬 쉽습니다.개인적으로 저는 데이터 분석을위한 컴퓨팅 소개를 마치고 프로그래밍 기술의 정점에있었습니다.가장 어려운 컴퓨터 공학 과정 인 데이터 및 시각적 분석을이 과정 직후에 수강했으면합니다.어떤 수업이 보완 과목으로 잘 어울리는 지 고려해야합니다.
OMSA 프로그램은 그만한 가치가 있습니까?
가치를 결정하려면 프로그램 비용과 프로그램 완료의 예상 수익을 추정해야합니다.이 프로그램의 금전적 비용은 13,000 달러 *이지만 프로그램을 완료하는 데 인건비는 2,160 시간 **입니다.비교 가능한 대학원 학위 측면 에서이 프로그램의 수업료는 스펙트럼의 가장 낮은 쪽입니다.그러나 사회에서 자격주의의 가치가 떨어지고 있다고 생각한다면이 자료를 배우는 데 훨씬 더 저렴한 옵션이 있습니다 (예 :Coursera,무료 온라인 리소스등).수업료가 당신과 관련이 없다면 시간 약속을해야합니다.앞서 언급했듯이이 프로그램은 깊이가 아닌 분석 공간의 폭에 관한 것입니다.실험, 인공 지능 또는 데이터 엔지니어링과 같은 분석 하위 집합의 전문가가되고 싶다면 다른 곳에서 시간을 보내는 것이 좋습니다.
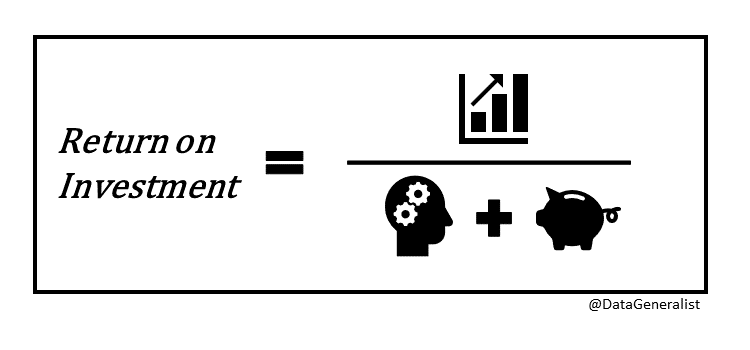
반환 관점에서이 프로그램은 주로 구조화 된 학습 환경과 최소한 엔트리 레벨 데이터 분석 위치의 두 가지를 제공합니다.이 프로그램은 각 분야의 전문가들이 제공하는 대부분의 과정에 대해 잘 짜여진 강의 계획서를 제공합니다.과제는 구성되어 있으며 일반적으로 가능한 한 적시에 피드백을받을 수 있도록 자동 채점자가 있습니다.환경에는 다음을 포함하여 과제에 대한 지원을 찾기위한 다양한 커뮤니케이션 모드가 포함됩니다광장, Slack 및 근무 시간 세션.특정 문제를 해결하기위한 힌트를 찾기 위해 Piazza의 수십 개의 게시물을 읽는 것은 약간 엉망 일 수 있습니다.이것은 그들이 당신의 학습을 가능하게하는 정도에 관한 것입니다.위의 어느 곳에서도 강의를 언급하지 않았 음을 알 수 있습니다.코스에 따라 맞거나 놓칠 수 있습니다.많은 강의는 수준이 너무 높아서 완전히 건너 뛰는 것이 현명 할 것입니다.일부 강의 또는 과제에는 유용한 링크가 포함됩니다.그러나 대부분의 학습은 자체 연구 (일명 인터넷 검색)를 통해 GT 환경 외부에서 이루어집니다.반환 관점에서이 프로그램에서 얻는 지식은 단순히 학위를 취득하는 것이 아니라 자료를 배우는 데 투입 한 노력에 크게 의존합니다.
대부분의 사람들이 대학원 프로그램에 등록하는 주된 이유 인 취업 기회로 이동합니다. 데이터 분석 기술에 대한 수요가 급증하고 있다는 점을 반복 할 필요가 없습니다. 모든 산업에서 기술에 대한 의존도가 높아짐에 따라 컴퓨터 과학과 통계 기술이 시장성이없는 미래는 보이지 않습니다. 인공 지능은 자동화 된 각 프로세스에 설치 및 지속적인 유지 관리가 필요하기 때문에 이러한 기술에 대한 우리의 의존도를 높일뿐입니다. 이 프로그램에는 너무 많은 유형의 개인이 등록되어 있기 때문에이 학위가 원하는 직업을 얻는 데 얼마나 도움이 될 것인지 말하기는 어렵습니다. 제 생각에는 OMSA 학위와 0 년의 업무 경험으로 졸업하는 학생은 3 ~ 5 년의 경력을 원하는 일반적인 분석 채용 공고에 적합합니다. 예를 들어 데이터 분석가, 선임 데이터 분석가, 비즈니스 인텔리전스 엔지니어, 주니어 데이터 과학자 및 데이터 과학 동료가 있습니다. 그러나 이러한 직책 중 하나를 최고의 회사 또는 더 고위 분석 역할에서 원하는 경우 추가 업무 경험이나 개인 프로젝트를 통해 학위를 늘려야합니다.
3 ~ 5 년의 추가 데이터 분석 경험을 추가하는 것이이 프로그램의 비용에 해당하는 가치가 있는지 여부는 귀하가 결정합니다.다음 기준 중 하나 이상에 해당하는 사람들에게이 프로그램이 가장 가치 있다고 생각합니다.
- 경력을 데이터 분석으로 전환하려는 정량적 / 프로그래밍 배경이있는 숙련 된 전문가
- 경험이 풍부한해석학3 ~ 5 년까지 전문적인 발전을 가속화하려는 전문가
- 학습을 용이하게하기위한 구조가 필요한 학생
- 끈기가 필요한 복잡한 문제를 두려워하지 않는 학생
- 특정 데이터 분석 또는 데이터 과학 주제 (예 : 인공 지능, 기계 학습 엔지니어링, 데이터 엔지니어링, 데이터 시각화 등)를 전문적으로 다루고 싶지 않은 숙련 된 전문가
* (학점당 275 시간 * 36 학점) + ((194 학점 + 학기당 107 학점) * 9 학기)
** (주당 3 학점당 12 시간 * 학기당 15 주) * (36/3 3 학점 블록)
이 프로그램을 어떻게 준비 할 수 있습니까?
이 프로그램에 지원하고 등록하기로 결정한 경우 자료에 대한 준비가되었는지 확인해야합니다.입학요구 사항학생들이이 프로그램을 준비 할 때 차선책입니다.이 프로그램에서 성공하려면 데이터 분석, 수학 및 컴퓨터 과학 전반에 걸쳐 일정 수준의 경험을 쌓는 것이 좋습니다.
데이터 분석 주제 및 도구익숙한와:
- SQL
- 뛰어나다
- 데이터 시각화
- 데이터 정리
- 데이터 분석
수학 측면에서 당신은편안다음과 함께 :
- 미적분-적분, 미분, 함수, 한계
- 통계 — 가설 검정, p- 값, 신뢰 구간, 샘플링
- 확률-분포, 오류
- 선형 대수 행렬, 행렬 연산, 벡터, 연립 방정식
컴퓨터 과학에 관한 한,이 프로그램에 앞서 두 개의 엄격한 학부 컴퓨터 과학 과정을 이수해야합니다.엄격함은 3 학점당 주당 10 시간 이상이 필요하다는 것을 의미합니다.적어도 하나의 객체 지향 프로그래밍 언어를 사용하는 중급 프로그래머 여야합니다.여기에는 코드 내 문제 디버깅 및 진단 경험이 포함됩니다.웹 개발에 대한 지식도 도움이 될 것입니다.무엇보다도 첫 번째 시도에서 거의 옳지 않은 어려운 문제를 해결하기위한 만족할 줄 모르는 욕구가 필요합니다.
추가 질문이나 의견이 있으면 언제든지밖.저는 항상 데이터 분석이나 금융에 대해 생각하고 있습니다.
Georgia Tech’s MS Analytics Program: My Review Part II
Georgia Tech’s MS Analytics Program: My Review Part II

After writing my first review of the OMSA program, I have been contacted by many prospective and current students. Because this program attracts students from a wide variety of backgrounds, providing advice that applies to everyone can be challenging. Below are some of the most common questions I receive and my attempt to answer them.
Is OMSA the right program for me?
If you read the program description on their degree overview page, they use the descriptor, “interdisciplinary”. I think this was the perfect word choice for the program. The range of topics covered in this program come from a wide variety of disciplines. The required courses cover finance, accounting, object-oriented programming, data analysis, machine learning, statistics, web development, cloud computing, data cleaning, scripting languages, and data visualization. The emphasis of this highly technical program is breadth over depth. If that sounds appealing, then this might be the program for you. However, if you wish to become an expert and specialize in any one of those individual disciplines, there are better options than this program.
Although this program emphasizes breadth, do not underestimate the difficulty level of the material. Being competent across business, computer science, and statistics is no easy feat. Often individuals will struggle with at least one of the disciplines covered in this program. If you are concerned with the technical rigor of the degree, I would advise you to improve your skills prior to enrollment or consider other programs. Other online quantitative master’s degrees include Data Science, Applied Economics, Statistics, Computer Science, Business Analytics, and an MBA: Analytics Concentration. Analytics programs run out of a university’s business school will likely be less rigorous in mathematics and computer science.
Which OMSA specialization should I choose?
After you finish the required courses, there are 3 specializations you can choose from. The word, specialization, is a bit misleading because the majority of the program is the same for every student. Only 6/36 credits are tailored to that specialization; otherwise, the rest is required or up to your discretion. You can quickly summarize the three specializations with the following:

Although the difficulty level is somewhat subjective, I think most students would agree with my assessment. The computer science courses tend to require the most hours of work per week.
Is there a particular order of classes that you would recommend?
With so many different disciplines covered in this program, it can be tricky to decide the appropriate order of classes to take. While deciding, there are many variables to take into account. Here are just a few of those questions to answer before you create your ideal 2 to 4-year graduate plan.
How many classes can you take per semester?
The average workload ranges from 8–20 hours per week per 3 credits, depending on the course. You need to consider which classes can be paired together based on the class expectations. Your individual time commitment will differ from the average depending on the strength of your skills in that subject. For example, if you have a strong background in statistics, then you can likely assume a below-average time commitment for statistics courses.
Which classes are offered each semester?
Summer semesters are shorter than Fall and Spring. Therefore, they only offer courses that can move at a quicker pace, which is a subset of the full class list. Because they run at a faster pace, the average workload per week is about 20 percent higher than average.
Which classes are optimal to take in close proximity?
It is much easier to take two related disciplines in close proximity to each other. Personally, I was at the peak of my programming skills after finishing Introduction for Computing for Data Analytics. I wish I took the hardest required computer science course, Data and Visual Analytics, immediately after this one. You should consider which classes pair well together as complementary subjects.
Is the OMSA program worth it?
To determine worth, you need to estimate the cost of the program and the expected return of completing it. The monetary cost for this program is 13,000 dollars*, but the labor cost is 2,160 hours** to complete the program. In terms of comparable graduate degrees, the tuition of this program is towards the lowest end of the spectrum. However, if you think the value of credentialism is waning in society, there are much cheaper options to learn this material (e.g. Coursera, Free online resources, etc.). If the cost of tuition doesn’t concern you the time commitment should. As stated previously, this program is about breadth in the analytics space rather than depth. If you wish to become an expert at a subset of analytics, such as experimentation, artificial intelligence, or data engineering, then your time is better spent elsewhere.
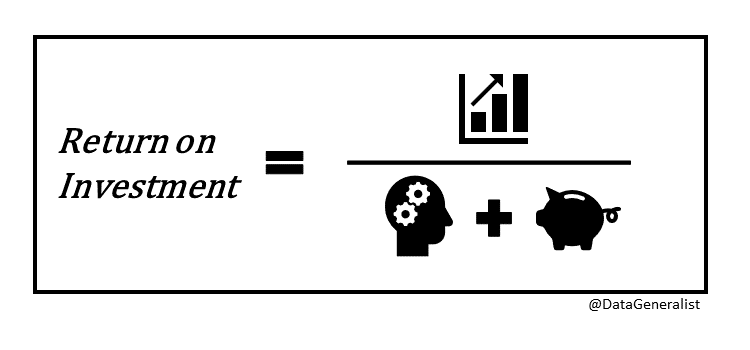
From a return perspective, the program primarily offers two things: a structured learning environment and, at a minimum, an entry-level data analytics position. The program offers a well-thought-out syllabus for most courses from experts in each of their respective domains. The assignments are organized and typically have auto-graders whenever possible for timely feedback. The environment includes multiple modes of communication to find assistance on assignments, including Piazza, Slack, and office hours sessions. Reading through dozens of posts on Piazza to find a hint at solving a particular problem can be a bit of a mess. This is about the extent to which they enable your learning. You will notice I did not mention lectures anywhere above. They can be hit or miss, depending on the course. Many lectures are so high level that you would be wiser to skip them entirely. Some lectures or assignments will include helpful links; however, the majority of your learning takes place outside of the GT environment through your own research (AKA Googling). As far as a return perspective, the knowledge you gain from this program is highly dependent upon the effort you put into learning the material versus simply getting a degree.
Moving onto the primary reason most people enroll in a graduate program, job opportunities. I don’t need to reiterate that data analytics skills are in hot demand. With increasing reliance on technology in every industry, I don’t see any future where computer science and statistics skills will not be marketable. Artificial intelligence will only increase our reliance on these skills because each automated process requires the installation and ongoing maintenance. Because there are so many types of individuals enrolled in this program, it is hard to say how much this degree will help you land the job you desire. In my opinion, a student graduating with an OMSA degree and zero years of work experience is qualified for your typical analytics job posting looking for 3–5 years of experience. Examples include data analysts, senior data analysts, business intelligence engineers, junior data scientists, and data science associates. However, if you want one of these positions at a top company or a more senior analytics role, you need to augment the degree with additional work experience or personal projects.
It’s up to you to decide whether adding the equivalent of an extra 3–5 years of data analytics experience is worth the cost of this program. I think the program is most valuable for those who fit at least one of the following criteria:
- An experienced professional with some quantitative/programming background looking to switch careers into data analytics
- An experienced analytics professional who wants to accelerate their professional advancement by 3–5 years
- A student who needs structure to facilitate their learning
- A student who is not afraid of complex problems that require persistence
- An experienced professional who does not want to specialize in a specific data analytics or data science subject (e.g. Artificial Intelligence, Machine Learning Engineering, Data Engineering, Data Visualization, etc.)
*(275 per credit hr * 36 credit hours) + ((194 + 107 fees per semester) * 9 semesters)
**(12 hours per week per 3 credits * 15 weeks per semester) * (36/3 three credit blocks)
How can I prepare for this program?
If you decide to apply and enroll in this program, you need to confirm you are prepared for the material. The admission requirements are suboptimal in preparing students for this program. In order to be successful in this program, I would recommend having a certain level of experience across data analytics, mathematics, and computer science.
Data analytics topics and tools you should be familiar with:
- SQL
- Excel
- Data visualization
- Data cleaning
- Data analysis
In terms of math, you should be comfortable with the following:
- Calculus- integrals, derivatives, functions, limits
- Statistics — hypothesis testing, p-values, confidence intervals, sampling
- Probability- distributions, error
- Linear Algebra- matrices, matrix operations, vectors, systems of equations
As far as computer science, you should have completed the equivalent of two, rigorous undergraduate computer science courses prior to this program. Rigorous means they required 10+ hours per week per 3 credits. You need to be an intermediate level programmer in at least one object-oriented programming language. This includes experience with debugging and diagnosing issues within code. Familiarity with web development will help, too. Most of all, you need an insatiable appetite for tackling difficult problems that will rarely be correct on the first attempt.
If you have any additional questions or comments, feel free to reach out. I am ALWAYS thinking about data analytics or finance.