2021년 1월 25일 모바일 게임 매출 순위
Rank | Game | Publisher |
---|---|---|
1 | 리니지2M | NCSOFT |
2 | 리니지M | NCSOFT |
3 | 기적의 검 | 4399 KOREA |
4 | 세븐나이츠2 | Netmarble |
5 | 메이플스토리M | NEXON Company |
6 | 라이즈 오브 킹덤즈 | LilithGames |
7 | Genshin Impact | miHoYo Limited |
8 | V4 | NEXON Company |
9 | 뮤 아크엔젤 | Webzen Inc. |
10 | Cookie Run: Kingdom | Devsisters Corporation |
11 | 블레이드&소울 레볼루션 | Netmarble |
12 | S.O.S:스테이트 오브 서바이벌 | KingsGroup Holdings |
13 | PUBG MOBILE | KRAFTON, Inc. |
14 | 리니지2 레볼루션 | Netmarble |
15 | KartRider Rush+ | NEXON Company |
16 | 미르4 | Wemade Co., Ltd |
17 | 바람의나라: 연 | NEXON Company |
18 | R2M | Webzen Inc. |
19 | 찐삼국 | ICEBIRD GAMES |
20 | FIFA ONLINE 4 M by EA SPORTS™ | NEXON Company |
21 | Lords Mobile: Kingdom Wars | IGG.COM |
22 | 블리치: 만해의 길 | DAMO NETWORK LIMITED |
23 | Roblox | Roblox Corporation |
24 | 라그나로크 오리진 | GRAVITY Co., Ltd. |
25 | A3: 스틸얼라이브 | Netmarble |
26 | Empires & Puzzles: Epic Match 3 | Small Giant Games |
27 | 명일방주 | Yostar Limited. |
28 | 그랑삼국 | YOUZU(SINGAPORE)PTE.LTD. |
29 | Pokémon GO | Niantic, Inc. |
30 | Epic Seven | Smilegate Megaport |
31 | Age of Z Origins | Camel Games Limited |
32 | AFK 아레나 | LilithGames |
33 | Gardenscapes | Playrix |
34 | Pmang Poker : Casino Royal | NEOWIZ corp |
35 | Brawl Stars | Supercell |
36 | 검은사막 모바일 | PEARL ABYSS |
37 | Top War: Battle Game | Topwar Studio |
38 | 한게임 포커 | NHN BIGFOOT |
39 | Cookie Run: OvenBreak – Endless Running Platformer | Devsisters Corporation |
40 | Homescapes | Playrix |
41 | FIFA Mobile | NEXON Company |
42 | 컴투스프로야구2021 | Com2uS |
43 | 검은강호2: 이터널 소울 | 9SplayDeveloper |
44 | 랑그릿사 | ZlongGames |
45 | 가디언 테일즈 | Kakao Games Corp. |
46 | 아일랜드M | Gamepub |
47 | Random Dice: PvP Defense | 111% |
48 | Summoners War | Com2uS |
49 | 카이로스 : 어둠을 밝히는 자 | Longtu Korea Inc. |
50 | 황제라 칭하라 | Clicktouch Co., Ltd. |
Create your first REST API in FastAPI -번역
FastAPI에서 첫 번째 REST API 만들기
Python에서 고성능 API를 만드는 단계별 가이드
이 포스트에서는FastAPI: Rest API를 생성하기위한 Python 기반 프레임 워크입니다.이 프레임 워크의 몇 가지 기본 기능을 간략하게 소개 한 다음 연락처 관리 시스템을위한 간단한 API 세트를 만들겠습니다.이 프레임 워크를 사용하려면 Python에 대한 지식이 매우 필요합니다.
FastAPI 프레임 워크를 논의하기 전에 REST 자체에 대해 조금 이야기 해 보겠습니다.
Wikipedia에서 :
REST (Representational State Transfer)웹 서비스를 만드는 데 사용할 제약 조건 집합을 정의하는 소프트웨어 아키텍처 스타일입니다.RESTful 웹 서비스라고하는 REST 아키텍처 스타일을 따르는 웹 서비스는 인터넷에서 컴퓨터 시스템 간의 상호 운용성을 제공합니다.RESTful 웹 서비스를 사용하면 요청 시스템이 일관되고 사전 정의 된 상태 비 저장 작업 집합을 사용하여 웹 리소스의 텍스트 표현에 액세스하고 조작 할 수 있습니다.SOAP 웹 서비스와 같은 다른 종류의 웹 서비스는 자체 임의의 작업 집합을 노출합니다. [1]
FastAPI 프레임 워크 란 무엇입니까?
공식에서웹 사이트:
FastAPI는 표준 Python 유형 힌트를 기반으로 Python 3.6 이상으로 API를 빌드하기위한 최신의 빠른 (고성능) 웹 프레임 워크입니다.
네, 그렇습니다빠른,매우 빠름ㅏ엔d 그것은의 상자 지원 때문입니다비동기의 특징Python 3.6 이상이것이 최신 버전의 Python을 사용하는 것이 권장되는 이유입니다.
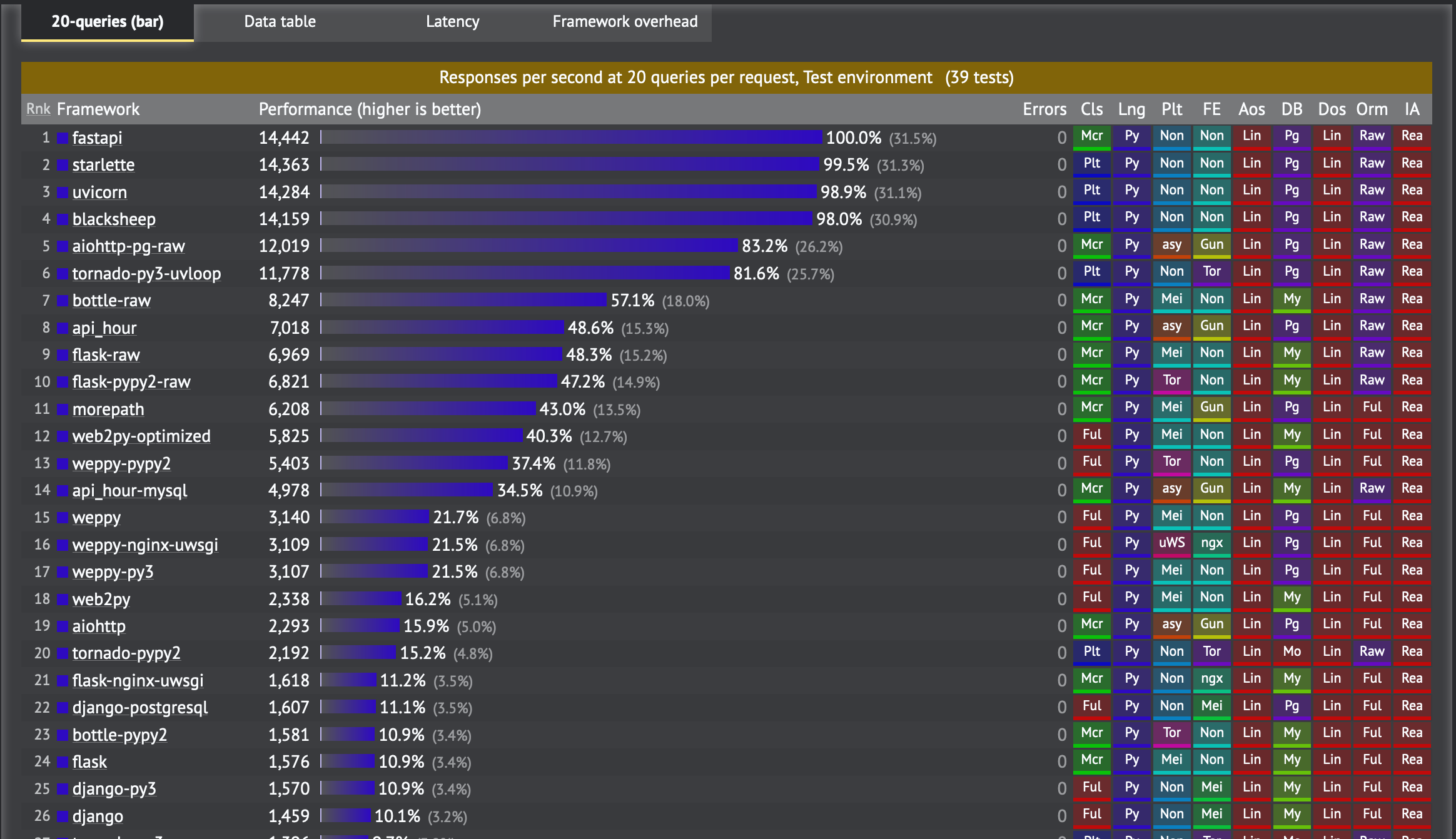
FastAPI는세바스티안 라미레스Flask 및 DRF와 같은 기존 프레임 워크에 만족하지 않았습니다.더 많은 것을 배울 수 있습니다여기.웹 사이트에 언급 된 주요 기능 중 일부는 다음과 같습니다.
- 빠른: 매우 높은 성능, 동급NodeJS과가다(Starlette와 Pydantic에게 감사드립니다).사용 가능한 가장 빠른 Python 프레임 워크 중 하나.
- 빠른 코딩: 기능 개발 속도를 약 200 % ~ 300 % 증가시킵니다.*
- 버그 감소: 인간 (개발자)이 유발하는 오류를 약 40 % 감소시킵니다.*
- 직관적: 훌륭한 편집기 지원.어디에서나 완성.디버깅 시간 단축.
- 쉬운: 사용하고 배우기 쉽도록 설계되었습니다.문서를 읽는 시간이 줄어 듭니다.
- 짧은: 코드 중복을 최소화합니다.각 매개 변수 선언의 여러 기능.더 적은 버그.
- 건장한: 프로덕션 준비 코드를 가져옵니다.자동 대화 형 문서.
- 표준 기반: API (Open API)에 대한 개방형 표준 기반 (완전히 호환 가능)
FastAPI의 제작자는 거인의 어깨에 서서 다음과 같은 기존 도구와 프레임 워크를 사용했다고 믿었습니다.Starlette과Pydantic
Installation and Setup
나는 사용할거야PipenvAPI 개발 환경을 설정합니다.Pipenv를 사용하면 컴퓨터에 설치된 항목에 관계없이 개발 환경을 쉽게 격리 할 수 있습니다.또한 컴퓨터에 설치된 것과 다른 Python 버전을 선택할 수 있습니다.그것은 사용합니다Pipfile
모든 프로젝트 관련 종속성을 관리합니다.여기서 Pipenv를 자세히 다루지 않을 것이므로 프로젝트에 필요한 명령 만 사용합니다.
다음을 실행하여 PyPy를 통해 Pipenv를 설치할 수 있습니다.pip 설치 pipenv
pipenv 설치 --python 3.9
설치가 완료되면 다음 명령을 실행하여 가상 환경을 활성화 할 수 있습니다.pipenv 쉘

당신은 또한 실행할 수 있습니다pipenv 설치 --three
여기서 3은 Python 3.x를 의미합니다.
설치가 완료되면 다음 명령을 실행하여 가상 환경을 활성화 할 수 있습니다.pipenv 쉘
먼저FastAPI다음 명령을 실행합니다.pipenv 설치 fastapi

그것은pipenv, 핍이 아닙니다.쉘에 들어가면 다음을 사용할 것입니다.pipenv
.기본적으로 pip를 사용하고 있지만 모든 항목은Pipfile
.그만큼Pipfile
아래와 같이 보일 것입니다.
이제 개발 환경을 설정했습니다.이제 첫 번째 API 엔드 포인트 작성을 시작할 때입니다.다음과 같은 파일을 생성하겠습니다.main.py
.이것이 우리 앱의 진입 점이 될 것입니다.
fastapi에서 가져 오기 FastAPI앱 = FastAPI ()@앱.가져 오기("/")
def home () :
return { "Hello": "FastAPI"}
Flask에서 작업 한 적이 있다면 거의 비슷할 것입니다.필요한 라이브러리를 가져온 후앱
인스턴스를 만들고 데코레이터로 첫 번째 경로를 만들었습니다.
이제 어떻게 실행하는지 궁금합니다.음, FastAPI는유비 콘ASGI 서버입니다.당신은 단순히 명령을 실행합니다uvicorn main : app --reload
파일 이름 (본관이 경우 .py) 및 클래스 객체 (앱이 경우) 서버를 시작합니다.나는-재 장전
변경 될 때마다 자동으로 다시로드되도록 플래그를 지정합니다.

방문http://localhost:8000/
JSON 형식으로 메시지가 표시됩니다.{ "Hello": "FastAPI"}
멋지죠?
FastAPI는 API 문서 엔진도 제공합니다.방문하면http://localhost:8000/docs
Swagger UI 인터페이스를 사용하고 있습니다.
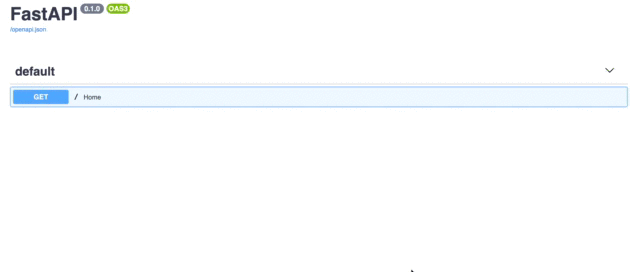
또는 멋진 것이 필요하면 방문하십시오http://localhost:8080/redoc

FastAPI는 다음과 같은 API 엔드 포인트의 OpenAPI 버전도 제공합니다.http://127.0.0.1:8000/openapi.json
Path and Parameters
앞으로 나아 갑시다.다른 API 엔드 포인트를 추가합니다.ID로 연락처 세부 정보를 가져 오는 것입니다.
@앱.get ( "/ contact / {contact_id}")
def contact_details (contact_id : int) :
{ 'contact_id': contact_id} 반환
여기에 방법이 있습니다.contact_details
그것은 단지 받아들입니다int
매개 변수를 그대로 반환합니다.dict
체재.이제 다음을 통해 액세스 할 때곱슬 곱슬하다다음과 같습니다.

이제 정수 대신 문자열을 전달하면 어떻게 될까요?아래 내용이 표시됩니다.

당신은 그것을 볼 않았다?잘못된 데이터 유형을 보냈다는 오류 메시지를 리턴했습니다.그런 사소한 일에 대해서는 유효성 검사기를 작성할 필요가 없습니다.그것이 일하는 동안의 아름다움입니다FaastAPI.
쿼리 문자열
쿼리 문자열 형태로 추가 데이터를 전달하면 어떻게됩니까?예를 들어 API 끝점은 많은 레코드를 반환하므로 페이지 매김이 필요합니다.음, 문제 없습니다. 해당 정보도 가져올 수 있습니다.
먼저선택 과목
유형:
import 입력에서
@app.get("/contact/{contact_id}")
def contact_details(contact_id: int, page: Optional[int] = 1):
if page:
return {'contact_id': contact_id, 'page': page}
return {'contact_id': contact_id}
여기에서 다른 매개 변수를 전달했습니다.페이지
유형을 설정선택 사항 [int]
여기.선택 사항이며 이름에서 알 수 있습니다.선택 과목매개 변수.유형 설정int
정수 값만 받아들이도록하는 것입니다. 그렇지 않으면 위에서했던 것처럼 오류가 발생합니다.
URL에 액세스http://127.0.0.1:8000/contact/1?page=5
다음과 같은 내용이 표시됩니다.

멋지죠?
지금까지 우리는 수동으로dict
.시원하지 않습니다.단일 값을 입력하고 YUUGE JSON 구조를 반환하는 것은 매우 일반적입니다.FastAPI는이를 처리하는 우아한 방법을 제공합니다.Pydantic 모델.
Pydantic모델은 실제로 데이터 유효성 검사에 도움이됩니다. 그게 무슨 뜻입니까?전달되는 데이터가 유효한지 확인하고 그렇지 않으면 오류를 반환합니다.우리는 이미 Python의 유형 힌트를 사용하고 있으며 이러한 데이터 모델은 삭제 된 데이터가 통과되도록합니다.약간의 코드를 작성해 봅시다.이 목적을 위해 연락처 API를 다시 확장하겠습니다.
import 입력에서fastapi에서 가져 오기 FastAPI
pydantic import BaseModel에서
앱 = FastAPI ()클래스 Contact (BaseModel) :
contact_id : int
first_name : str
last_name : str
user_name : str
암호 : str@앱.post ( '/ 연락처')
async def create_contact (연락처 : 연락처) :
반환 연락처
나는 수입했다BaseModel
pydantic의 클래스.그 후, 저는BaseModel
클래스에 3 개의 필드를 설정합니다.나는 또한 그것의 유형을 설정하고 있음을 주목하십시오.완료되면우편
API 끝점 및접촉
그것에 매개 변수.나는 또한 사용하고 있습니다비동기
여기에 간단한 파이썬 함수를코 루틴.FastAPI는 즉시 지원합니다.
이동http://localhost:8080/docs
다음과 같은 내용이 표시됩니다.

CURL 명령을 실행하면 다음과 같은 내용이 표시됩니다.

예상대로 방금 반환접촉
JSON 형식의 개체.
아시다시피 암호를 포함하여 전체 모델을 JSON 형식으로 덤프합니다.암호가 일반 텍스트 형식이 아니더라도 의미가 없습니다.그래서 뭐 할까?응답 모델답입니다.
응답 모델이란?
이름에서 알 수 있듯이응답 모델요청에 대한 응답을 보내는 동안 사용되는 모델입니다.기본적으로 방금 모델을 사용하면 모든 필드를 반환합니다.응답 모델을 사용하여 사용자에게 반환 할 데이터의 종류를 제어 할 수 있습니다.코드를 약간 변경해 보겠습니다.
클래스 Contact (BaseModel) :
contact_id : int
first_name : str
last_name : str
user_name : str
암호 : strContactOut (BaseModel) 클래스 :
contact_id : int
first_name : str
last_name : str
user_name : str@앱.가져 오기("/")
def home () :
return { "Hello": "FastAPI"}@앱.post ( '/ contact', response_model = ContactOut)
async def create_contact (연락처 : 연락처) :
반환 연락처
다른 수업을 추가했습니다.ContactOut
거의 사본입니다접촉
수업.여기서 다른 점은암호
들.그것을 사용하기 위해, 우리는 그것을response_model
매개 변수우편데코레이터.그게 다야.이제 동일한 URL을 실행하면 암호 필드가 반환되지 않습니다.

보시다시피 여기에는 비밀번호 필드가 표시되지 않습니다.눈치 채면/ docs
URL도 볼 수 있습니다.
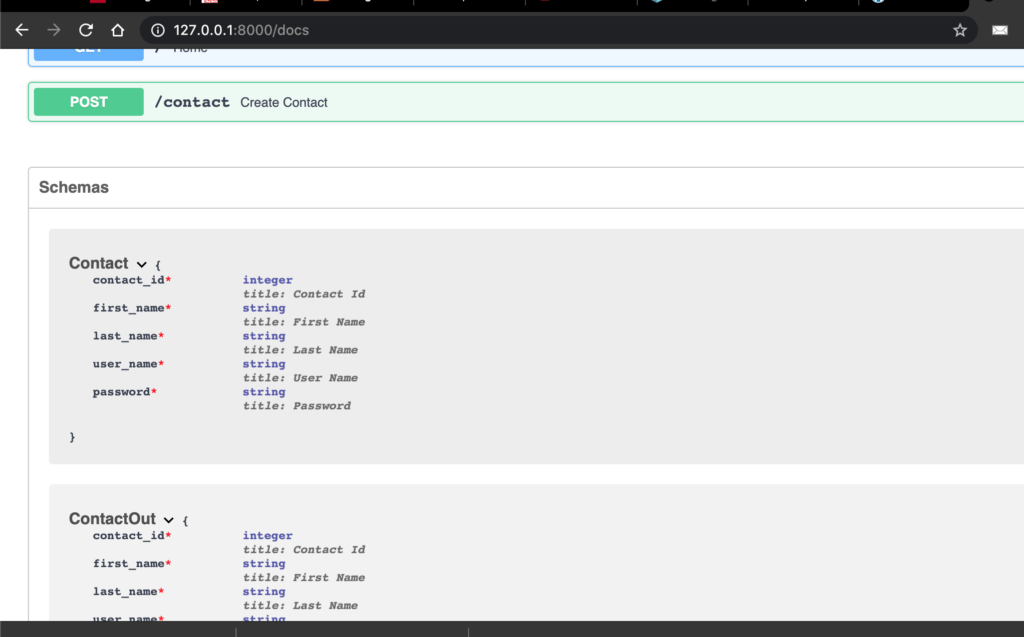
여러 방법으로 사용하려는 경우 다른 응답 모델을 사용하는 것이 가능하지만 단일 방법에서 기밀 정보를 생략하려는 경우에는 다음을 사용할 수도 있습니다.response_model_exclude
데코레이터의 매개 변수.
@앱.post ( '/ contact', response_model = 연락처, response_model_exclude = { "password"})
async def create_contact (연락처 : 연락처) :
반환 연락처
출력은 비슷합니다.당신은response_model
과response_model_exclude
여기.결과는 동일합니다.API 엔드 포인트에 메타 데이터를 연결할 수도 있습니다.
@앱.post ( '/ contact', response_model = 연락처, response_model_exclude = { "password"}, description = "단일 연락처 만들기")
async def create_contact (연락처 : 연락처) :
반환 연락처
문서에서 볼 수있는이 엔드 포인트에 대한 설명을 추가했습니다.

FastAPI 문서의 우수성은 여기서 끝나지 않으며 모델의 예제 JSON 구조를 설정할 수도 있습니다.
클래스 Contact (BaseModel) :
contact_id : int
first_name : str
last_name : str
user_name : str
암호 : str클래스 구성 :
schema_extra = {
"예": {
"contact_id": 1,
"first_name": "존",
"last_name": "미상",
"user_name": "jhon_123",
}
}
그렇게하면 다음과 같이 렌더링됩니다.

FastAPI에서 오류 처리
필요한 정보를 얻지 못할 수도 있습니다.FastAPI는HTTPException
그러한 상황을 다루는 수업.
@앱.get ( "/ contact / {id}", response_model = Contact, response_model_exclude = { "password"}, description = "단일 연락처 가져 오기")
async def contact_details (id : int) :
ID & lt;1:
HTTPException (status_code = 404, detail = "필요한 연락처 정보를 찾을 수 없음")
연락처 = 연락처 (contact_id = id, first_name = 'Adnan', last_name = 'Siddiqi', user_name = 'adnan1', password = 'adn34')
반환 연락처
A simple endpoint. It returns contact details based on the id. If the 신분증
1보다 작 으면 a를 반환합니다.404세부 사항이있는 오류 메시지.
떠나기 전에 사용자 지정 헤더를 보내는 방법을 알려 드리겠습니다.
fastapi에서 가져 오기 FastAPI, HTTPException, 응답@앱.get ( "/ contact / {id}", response_model = 연락처, response_model_exclude = { "password"},
description = "단일 연락처 가져 오기")
async def contact_details (id : int, response : Response) :
response.headers["X-LOL"] = "1"
ID & lt;1:
HTTPException (status_code = 404, detail = "필요한 연락처 정보를 찾을 수 없음")
연락처 = 연락처 (contact_id = id, first_name = 'Adnan', last_name = 'Siddiqi', user_name = 'adnan1', password = 'adn34')
반환 연락처
가져온 후Response
내가 통과 한 수업의뢰
유형의 매개 변수의뢰
헤더를 설정X-LOL
After running the curl command you will see something like the below:

You can find x-lol
헤더 중.LOL!
Conclusion
따라서이 게시물에서는 고성능 API를 빌드하기 위해 FastAPI를 사용하는 방법을 배웠습니다.우리는 이미 Flask라는 최소한의 프레임 워크를 가지고 있지만 FastAPI의 비동기 지원은 REST API를 통해 액세스되는 기계 학습 모델과 같은 최신 프로덕션 시스템에 매우 매력적입니다.나는 그 표면을 긁었다.자세한 내용은official FastAPI웹 사이트.
다음 포스트에서는 DB와의 통합, 인증 등과 같은 몇 가지 고급 주제에 대해 논의 할 것입니다.
Originally published at http://blog.adnansiddiqi.me on January 23, 2021.
Create your first REST API in FastAPI
Create your first REST API in FastAPI
A step by step guide creating high performance APIs in Python
In this post, I am going to introduce FastAPI: A Python-based framework to create Rest APIs. I will briefly introduce you to some basic features of this framework and then we will create a simple set of APIs for a contact management system. Knowledge of Python is very necessary to use this framework.
Before we discuss the FastAPI framework, let’s talk a bit about REST itself.
From Wikipedia:
Representational state transfer (REST) is a software architectural style that defines a set of constraints to be used for creating Web services. Web services that conform to the REST architectural style, called RESTful Web services, provide interoperability between computer systems on the Internet. RESTful Web services allow the requesting systems to access and manipulate textual representations of Web resources by using a uniform and predefined set of stateless operations. Other kinds of Web services, such as SOAP Web services, expose their own arbitrary sets of operations.[1]
What is the FastAPI framework?
From the official website:
FastAPI is a modern, fast (high-performance), web framework for building APIs with Python 3.6+ based on standard Python type hints.
Yes, it is fast, very fast and it is due to out of the box support of the async feature of Python 3.6+ this is why it is recommended to use the latest versions of Python.
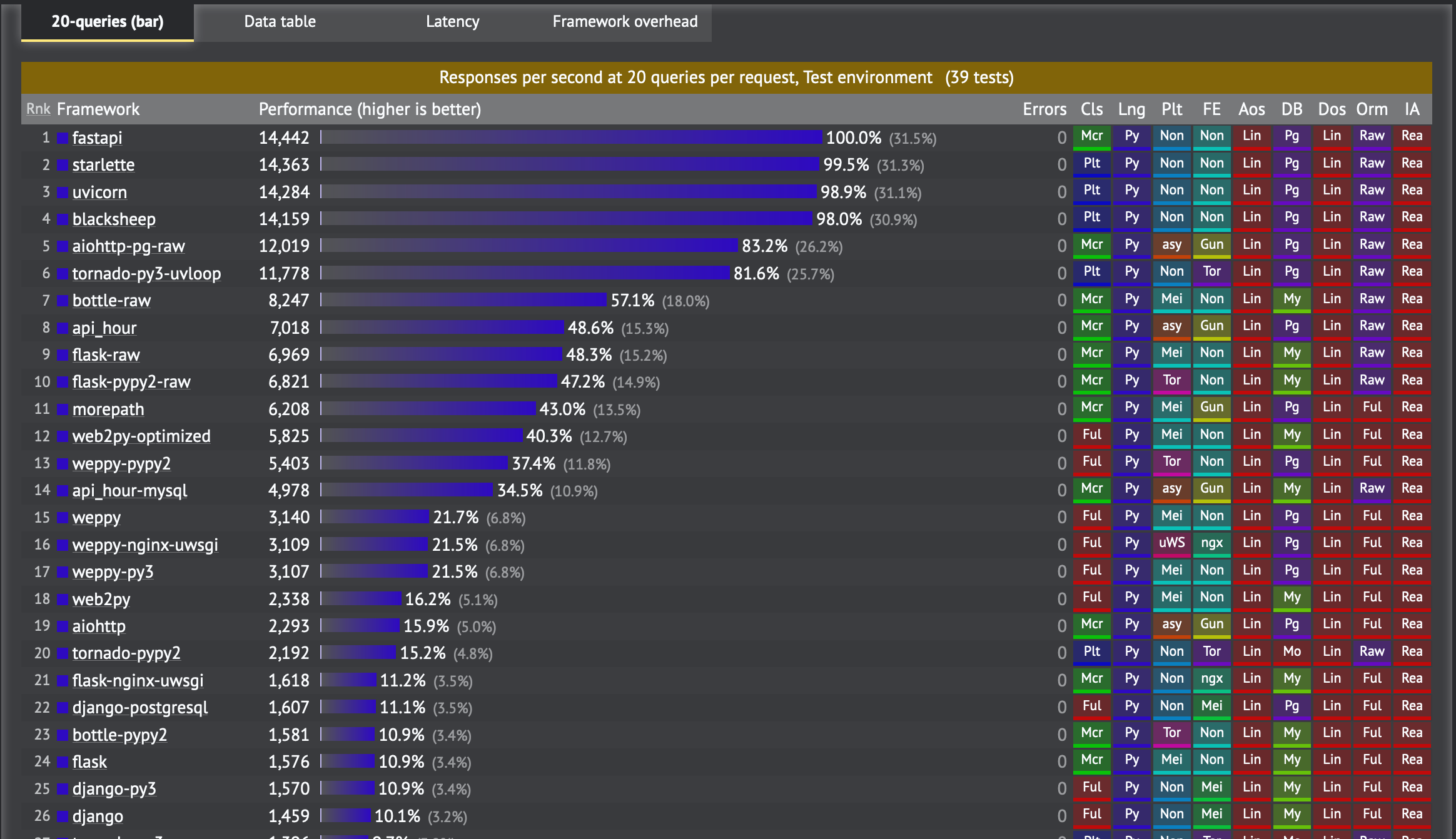
FastAPI was created by Sebastián Ramírez who was not happy with the existing frameworks like Flask and DRF. More you can learn about it here. Some of the key features mentioned on their website are:
- Fast: Very high performance, on par with NodeJS and Go (thanks to Starlette and Pydantic). One of the fastest Python frameworks available.
- Fast to code: Increase the speed to develop features by about 200% to 300%. *
- Fewer bugs: Reduce about 40% of human (developer) induced errors. *
- Intuitive: Great editor support. Completion everywhere. Less time debugging.
- Easy: Designed to be easy to use and learn. Less time reading docs.
- Short: Minimize code duplication. Multiple features from each parameter declaration. Fewer bugs.
- Robust: Get production-ready code. With automatic interactive documentation.
- Standards-based: Based on (and fully compatible with) the open standards for APIs(Open API)
The creator of the FastAPI believed in standing on the shoulder of giants and used existing tools and frameworks like Starlette and Pydantic
Installation and Setup
I am going to use Pipenv for setting up the development environment for our APIs. Pipenv makes it easier to isolate your development environment irrespective of what things are installed on your machine. It also lets you pick a different Python version than whatever is installed on your machine. It uses Pipfile
to manage all your project-related dependencies. I am not gonna cover Pipenv here in detail so will only be using the commands that are necessary for the project.
You can install Pipenv via PyPy by running pip install pipenv
pipenv install --python 3.9
Once installed you can activate the virtual environment by running the command pipenv shell

You may also run pipenv install --three
where three means Python 3.x.
Once installed you can activate the virtual environment by running the command pipenv shell
First, we will install FastAPI by running the following command: pipenv install fastapi

Note it’s pipenv, NOT pip. When you are into the shell you will be using pipenv
. Underlying it is using pip but all entries are being stored in Pipfile
. The Pipfile
will be looking like below:
OK, so we have set up our dev environment. It’s time to start writing our first API endpoint. I am going to create a file called main.py
. This will be the entry point of our app.
from fastapi import FastAPIapp = FastAPI()@app.get("/")
def home():
return {"Hello": "FastAPI"}
If you have worked on Flask then you will be finding it pretty much similar. After importing the required library you created an app
instance and created your first route with a decorator.
Now you wonder how to run it. Well, FastAPI comes with the uvicorn which is an ASGI server. You will simply be running the command uvicorn main:app --reload
You provide the file name(main.py in this case) and the class object(app in this case) and it will initiate the server. I am using the --reload
flag so that it reloads itself after every change.

Visit http://localhost:8000/
and you will see the message in JSON format {"Hello":"FastAPI"}
Cool, No?
FastAPI provides an API document engine too. If you visit http://localhost:8000/docs
which is using the Swagger UI interface.
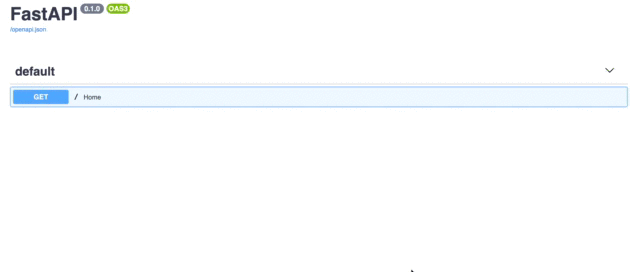
Or if you need something fancy then visit http://localhost:8080/redoc

FastAPI also provides an OpenAPI version of API endpoints, like this http://127.0.0.1:8000/openapi.json
Path and Parameters
Let’s move forward. We add another API endpoint. Say, it’s about fetching contact details by its id.
@app.get("/contact/{contact_id}")
def contact_details(contact_id: int):
return {'contact_id': contact_id}
So here is a method, contact_details
that accepts only an int
parameter and just returns it as it in a dict
format. Now when I access it via cURL it looks like below:

Now, what if I pass a string instead of an integer? You will see the below

Did you see it? it returned an error message that you sent the wrong data type. You do not need to write a validator for such petty things. That’s the beauty while working in FaastAPI.
Query String
What if you pass extra data in the form of query strings? For instance your API end-point returns loads of records hence you need pagination. Well, no issue, you can fetch that info as well.
First, we will import the Optional
type:
from typing import Optional
@app.get("/contact/{contact_id}")
def contact_details(contact_id: int, page: Optional[int] = 1):
if page:
return {'contact_id': contact_id, 'page': page}
return {'contact_id': contact_id}
Here, I passed another parameter, page
and set its type Optional[int]
here. Optional, well as the name suggests it’s an optional parameter. Setting the type int
is making sure that it only accepts the integer value otherwise, it’d be throwing an error like it did above.
Access the URL http://127.0.0.1:8000/contact/1?page=5
and you will see something like below:

Cool, No?
So far we just manually returned the dict
. It is not cool. It is quite common that you input a single value and return a YUUGE JSON structure. FastAPI provides an elegant way to deal with it, using Pydantic models.
Pydantic models actually help in data validation, what does it mean? It means it makes sure that the data which is being passed is valid, if not otherwise it returns an error. We are already using Python’s type hinting and these data models make that the sanitized data is being passed thru. Let’s write a bit of code. I am again going to extend the contact API for this purpose.
from typing import Optionalfrom fastapi import FastAPI
from pydantic import BaseModel
app = FastAPI()class Contact(BaseModel):
contact_id:int
first_name:str
last_name:str
user_name:str
password:str@app.post('/contact')
async def create_contact(contact: Contact):
return contact
I imported the BaseModel
class from pydantic. After that, I created a model class that extended the BaseModel
class and set 3 fields in it. Do notice I am also setting the type of it. Once it’s done I created a POST
API endpoint and passed an Contact
parameter to it. I am also using async
here which converts the simple python function into a coroutine. FastAPI supports it out of the box.
Go to http://localhost:8080/docs
and you will see something like below:

When you run the CURL command you would see something like the below:

As expected it just returned the Contact
object in JSON format.
As you notice, it just dumps the entire model in JSON format, including password. It does not make sense even if your password is not in plain-text format. So what to do? Response Model is the answer.
What is Response Model
As the name suggests, a Response Model is a model that is used while sending a response against a request. Basically, when you just used a model it just returns all fields. By using a response model you can control what kind of data should be returned back to the user. Let’s change the code a bit.
class Contact(BaseModel):
contact_id:int
first_name:str
last_name:str
user_name:str
password:strclass ContactOut(BaseModel):
contact_id:int
first_name:str
last_name:str
user_name:str@app.get("/")
def home():
return {"Hello": "FastAPI"}@app.post('/contact', response_model=ContactOut)
async def create_contact(contact: Contact):
return contact
I have added another class, ContactOut
which is almost a copy of the Contact
class. The only thing which is different here is the absence of the password
field. In order to use it, we are going to assign it in the response_model
parameter of the post decorator. That’s it. Now when I run hit the same URL it will not return the password field.

As you can see, no password field is visible here. If you notice the /docs
URL you will see it visible over there as well.
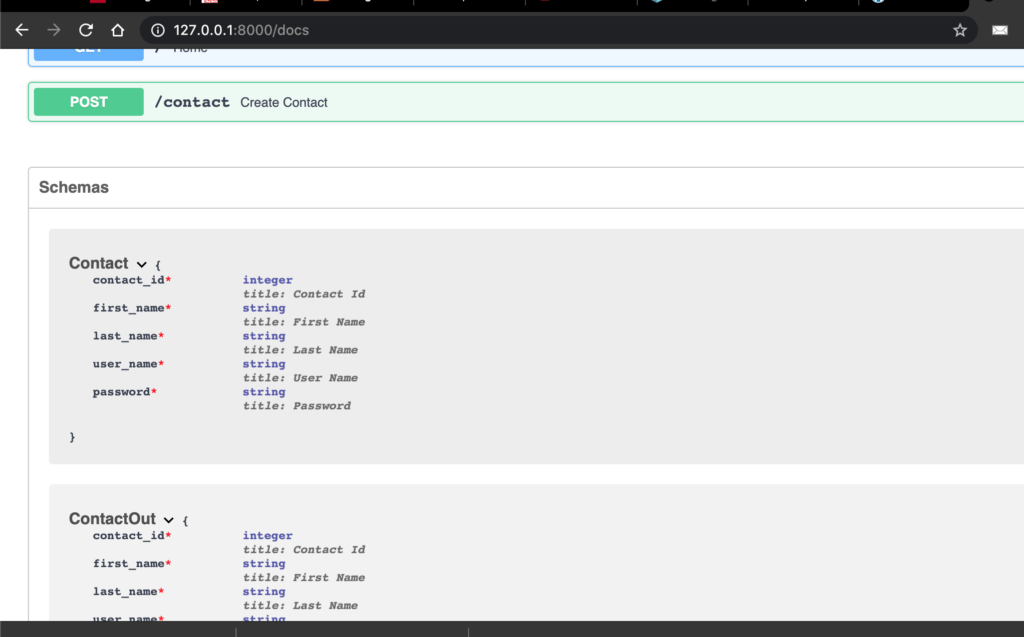
Using a different Response Model is feasible if you are willing to use it in multiple methods but if you just want to omit the confidential information from a single method then you can also use response_model_exclude
parameter in the decorator.
@app.post('/contact', response_model=Contact, response_model_exclude={"password"})
async def create_contact(contact: Contact):
return contact
The output will be similar. You are setting the response_model
and response_model_exclude
here. The result is the same. You can also attach metadata with your API endpoint.
@app.post('/contact', response_model=Contact, response_model_exclude={"password"},description="Create a single contact")
async def create_contact(contact: Contact):
return contact
We added the description of this endpoint which you can see in the doc.

FastAPI documentation awesomeness does not end here, it also lets you set the example JSON structure of the model.
class Contact(BaseModel):
contact_id:int
first_name:str
last_name:str
user_name:str
password:strclass Config:
schema_extra = {
"example": {
"contact_id": 1,
"first_name": "Jhon",
"last_name": "Doe",
"user_name": "jhon_123",
}
}
And when you do that, it is rendered as:

Error handling in FastAPI
It is always possible that you do not get the required info. FastAPI provides HTTPException
class to deal with such situations.
@app.get("/contact/{id}", response_model=Contact, response_model_exclude={"password"},description="Fetch a single contact")
async def contact_details(id: int):
if id < 1:
raise HTTPException(status_code=404, detail="The required contact details not found")
contact = Contact(contact_id=id, first_name='Adnan', last_name='Siddiqi', user_name='adnan1', password='adn34')
return contact
A simple endpoint. It returns contact details based on the id. If the id
is less than 1 it returns a 404 error message with details.
Before I leave, let me tell you how you can send custom headers.
from fastapi import FastAPI, HTTPException, Response@app.get("/contact/{id}", response_model=Contact, response_model_exclude={"password"},
description="Fetch a single contact")
async def contact_details(id: int, response: Response):
response.headers["X-LOL"] = "1"
if id < 1:
raise HTTPException(status_code=404, detail="The required contact details not found")
contact = Contact(contact_id=id, first_name='Adnan', last_name='Siddiqi', user_name='adnan1', password='adn34')
return contact
After importing the Response
class I passed request
parameter of type Request
and set the header X-LOL
After running the curl command you will see something like the below:

You can find x-lol
among headers. LOL!
Conclusion
So in this post, you learned how you can start using FastAPI for building high-performance APIs. We already have a minimal framework called Flask but FastAPI’s asynchronous support makes it much attractive for modern production systems especially machine learning models that are accessed via REST APIs. I have only scratched the surface of it. You can learn further about it on the official FastAPI website.
Hopefully, in the next post, I will be discussing some advanced topics like integrating with DB, Authentication, and other things.
Originally published at http://blog.adnansiddiqi.me on January 23, 2021.
2021년 1월 24일 모바일 게임 매출 순위
Rank | Game | Publisher |
---|---|---|
1 | 리니지2M | NCSOFT |
2 | 리니지M | NCSOFT |
3 | 기적의 검 | 4399 KOREA |
4 | 세븐나이츠2 | Netmarble |
5 | 메이플스토리M | NEXON Company |
6 | 라이즈 오브 킹덤즈 | LilithGames |
7 | Genshin Impact | miHoYo Limited |
8 | V4 | NEXON Company |
9 | 뮤 아크엔젤 | Webzen Inc. |
10 | 블레이드&소울 레볼루션 | Netmarble |
11 | S.O.S:스테이트 오브 서바이벌 | KingsGroup Holdings |
12 | PUBG MOBILE | KRAFTON, Inc. |
13 | KartRider Rush+ | NEXON Company |
14 | 미르4 | Wemade Co., Ltd |
15 | 리니지2 레볼루션 | Netmarble |
16 | 바람의나라: 연 | NEXON Company |
17 | R2M | Webzen Inc. |
18 | 찐삼국 | ICEBIRD GAMES |
19 | FIFA ONLINE 4 M by EA SPORTS™ | NEXON Company |
20 | Cookie Run: Kingdom | Devsisters Corporation |
21 | Lords Mobile: Kingdom Wars | IGG.COM |
22 | A3: 스틸얼라이브 | Netmarble |
23 | Roblox | Roblox Corporation |
24 | 블리치: 만해의 길 | DAMO NETWORK LIMITED |
25 | 라그나로크 오리진 | GRAVITY Co., Ltd. |
26 | Empires & Puzzles: Epic Match 3 | Small Giant Games |
27 | 명일방주 | Yostar Limited. |
28 | 그랑삼국 | YOUZU(SINGAPORE)PTE.LTD. |
29 | Pokémon GO | Niantic, Inc. |
30 | Epic Seven | Smilegate Megaport |
31 | Age of Z Origins | Camel Games Limited |
32 | AFK 아레나 | LilithGames |
33 | Pmang Poker : Casino Royal | NEOWIZ corp |
34 | Brawl Stars | Supercell |
35 | 검은사막 모바일 | PEARL ABYSS |
36 | Gardenscapes | Playrix |
37 | Top War: Battle Game | Topwar Studio |
38 | Cookie Run: OvenBreak – Endless Running Platformer | Devsisters Corporation |
39 | 한게임 포커 | NHN BIGFOOT |
40 | Homescapes | Playrix |
41 | FIFA Mobile | NEXON Company |
42 | 컴투스프로야구2021 | Com2uS |
43 | 아일랜드M | Gamepub |
44 | Random Dice: PvP Defense | 111% |
45 | 랑그릿사 | ZlongGames |
46 | 가디언 테일즈 | Kakao Games Corp. |
47 | 황제라 칭하라 | Clicktouch Co., Ltd. |
48 | 카이로스 : 어둠을 밝히는 자 | Longtu Korea Inc. |
49 | Summoners War | Com2uS |
50 | Rise of Empires: Ice and Fire | Long Tech Network Limited |
Modern Recommender Systems -번역
최신 추천 시스템
Facebook 및 Google과 같은 회사가 비즈니스를 구축 한 AI 알고리즘에 대해 자세히 알아보십시오.
최근 2019 년 5 월에 Facebook은 몇 가지 추천 접근 방식을 오픈 소스로 제공하고DLRM(딥 러닝 추천 모델).이 블로그 게시물은 DLRM 및 기타 최신 추천 접근 방식이 도메인의 이전 결과에서 파생 될 수있는 방법을 살펴보고 내부 작동 및 직관을 자세히 설명함으로써 어떻게 그리고 왜 잘 작동하는지 설명하기위한 것입니다.
맞춤형 AI 기반 광고는 요즘 온라인 마케팅에서 게임의 이름은 Facebook, Google, Amazon, Netflix 및 co와 같은 회사가 온라인 마케팅 정글의 왕입니다. 왜냐하면 이러한 추세를 채택했을뿐만 아니라 본질적으로이를 발명하고 전체 비즈니스 전략을 구축했기 때문입니다.그것.넷플릭스의“즐길 수있는 다른 영화”나 아마존의“이 상품을 구매 한 고객도 구매했습니다…”는 온라인 세상에서 많은 예입니다.
자연스럽게, 저는 일상적인 Facebook과 Gooogle 사용자로서 어느 시점에서 스스로에게 물었습니다.
“이게 정확히 어떻게 작동합니까?”
예, 우리 모두는 협업 필터링 / 행렬 분해가 작동하는 방식을 설명하는 기본적인 영화 추천 예제를 알고 있습니다.또한, 사용자가 특정 제품을 좋아하는지 여부에 대한 확률을 출력하는 간단한 분류기를 사용자별로 훈련시키는 접근 방식에 대해 말하는 것이 아닙니다.이 두 가지 접근 방식, 즉 협업 필터링과 콘텐츠 기반 추천은 사용할 수있는 성능과 예측을 산출해야하지만 Google, Facebook 및 Co는 확실히 더 나은 것을 가져야합니다. 그렇지 않으면 어디에 있지 않을 것입니다.그들은 오늘입니다.
오늘날의 고급 추천 시스템의 출처를 이해하려면 문제에 대한 두 가지 기본 접근 방식을 살펴보아야합니다.
특정 사용자가 특정 항목을 얼마나 좋아하는지 예측합니다.
온라인 마케팅 세계에서는 평점, 좋아요 등과 같은 명시적인 피드백과 클릭, 검색 기록, 댓글 또는 같은 암시 적 피드백을 기반으로 가능한 광고의 클릭률 (CTR)을 예측하는 데 추가됩니다.웹 사이트 방문.
콘텐츠 기반 필터링과협업필터링
1. 콘텐츠 기반 필터링
느슨하게 말한 콘텐츠 기반 추천은 사용자의 온라인 기록을 사용하여 사용자가 특정 제품을 좋아하는지 여부를 예측하는 것을 의미합니다.여기에는 사용자가 제공 한 좋아요 (예 : Facebook), 검색 한 키워드 (예 : Google), 단순히 특정 웹 사이트를 클릭하고 방문한 횟수가 포함됩니다.대체로 사용자의 선호도에 초점을 맞 춥니 다.예를 들어이 사용자의 특정 광고 그룹에 대한 클릭률 (또는 등급)을 출력하는 간단한 이진 분류기 (또는 회귀 자)를 생각할 수 있습니다.
2. 협업 필터링
그러나 협업 필터링은 유사한 사용자의 선호도를 조사하여 사용자가 특정 제품을 좋아할지 여부를 예측하려고합니다.여기에서 등급 매트릭스가 사용자와 영화에 대한 하나의 임베딩 매트릭스로 분해되는 영화 추천에 대한 표준 매트릭스 분해 (MF) 접근 방식을 생각할 수 있습니다.
클래식 MF의 단점은 예를 들어 어떤 부가 기능도 사용할 수 없다는 것입니다.영화 장르, 개봉일 등 MF 자체는 기존의 상호 작용을 통해 배워야합니다.또한 MF는 아직 누구에게도 평가되지 않은 신작은 추천 할 수없는 이른바 ‘콜드 스타트 문제’에 시달리고있다.콘텐츠 기반 필터링은 이러한 두 가지 문제를 해결하지만 유사한 사용자의 선호도를 볼 수있는 예측 능력이 부족합니다.
두 가지 다른 접근 방식의 장점과 단점은 두 아이디어가 어떻게 든 하나의 모델로 결합되는 하이브리드 접근 방식의 필요성을 매우 분명하게 나타냅니다.
하이브리드 추천 모델
1. 분해 기계
2010 년 Steffen Rendle이 소개 한 아이디어 중 하나는분해 기계.행렬 분해와 회귀를 결합하는 기본적인 수학적 접근 방식을 보유합니다.
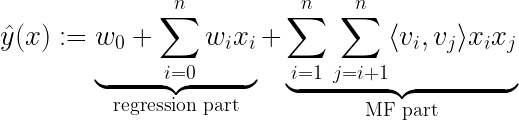
학습 중에 추정해야하는 모델 매개 변수는 다음과 같습니다.

⟨∙, ∙⟩은 V에서 행으로 볼 수있는 ℝᵏ 크기의 vᵢ와 vⱼ 두 벡터 사이의 내적입니다.
이 모델에 던져지는 데이터 x를 표현하는 방법의 예를 볼 때이 방정식이 어떻게 의미가 있는지 보는 것은 매우 간단합니다.Steffen Rendle의 Factorization Machines에 대한 백서에 설명 된 예제를 살펴 보겠습니다.
사용자가 특정 시간에 영화에 등급을 부여하는 영화 리뷰에 다음과 같은 거래 데이터가 있다고 상상해보십시오.
- user u ∈ U = {Alice (A), Bob (B), . . .}
- movie (item) i ∈ I = {Titanic (TI), Notting Hill (NH), Star Wars (SW), Star Trek (ST), . . .}
- rating r ∈ {1,2,3,4,5} at time t ∈ ℝ

위의 그림을 보면 하이브리드 추천 모델에 대한 데이터 설정을 볼 수 있습니다.사용자와 항목을 나타내는 희소 특성과 추가 메타 또는 부가 정보 (예 :이 예에서 “시간”또는 “최근 영화 등급”)는 모두 대상 y에 매핑되는 특성 벡터 x의 일부입니다.이제 핵심은 모델에 의해 처리되는 방법입니다.
- FM의 회귀 부분은 표준 회귀 작업과 같이 희소 데이터 (예 : “사용자”)와 고밀도 데이터 (예 : “시간”)를 모두 처리하므로 FM 내에서 콘텐츠 기반 필터링 접근 방식으로 해석 될 수 있습니다.
- FM의 MF 부분은 이제 기능 블록 간의 상호 작용 (예 : “사용자”와 “영화”간의 상호 작용)을 설명합니다. 여기서 행렬 V는 협업 필터링 접근 방식에 사용되는 임베딩 행렬로 해석 될 수 있습니다.이러한 교차 사용자 영화 관계는 다음과 같은 통찰력을 제공합니다.
vⱼ를 포함하는 다른 사용자 j와 유사한 임베딩 vᵢ (영화 속성에 대한 선호도를 나타냄)를 가진 사용자 i는 사용자 j와 유사한 영화를 매우 좋아할 수 있습니다.
회귀 부분과 MF 부분에 대한 두 가지 예측을 함께 추가하고 하나의 비용 함수에서 매개 변수를 동시에 학습하면 이제 사용자를위한 권장 사항을 만들기 위해 “양쪽 세계의 최고”접근 방식을 사용하는 하이브리드 FM 모델이 생성됩니다.
언뜻보기에 Factorization Machine의이 하이브리드 접근 방식은 이미 NLP 또는 컴퓨터 비전과 같은 많은 다른 AI 분야가 과거에 입증 되었 듯이 완벽한 “양쪽 세계의 최고”모델 인 것처럼 보입니다.
“신경망에 던져 넣으면 더 나아질 것입니다.”
2. 넓고 깊은 NCF (Neural Collaborative Filtering) 및 DeepFM (Deep Factorization Machine)
먼저 NCF 논문을 살펴봄으로써 신경망 접근 방식으로 협업 필터링을 어떻게 해결할 수 있는지 살펴볼 것입니다. 이는 분해 기계의 신경망 버전 인 Deep Factorization Machines (DeepFM)로 이어질 것입니다.왜 그들이 일반 FM보다 우월하고 신경망 아키텍처를 해석 할 수 있는지 알아볼 것입니다.추천 시스템에서 딥 러닝의 첫 번째 주요 혁신 중 하나 인 Google에서 이전에 출시 한 Wide & amp; Deep 모델을 개선하여 DeepFM이 어떻게 개발되었는지 살펴 보겠습니다.이것은 마침내 우리를 DeepFM에 대한 단순화 및 약간의 조정으로 볼 수있는 2019 년 Facebook에서 발표 한 앞서 언급 한 DLRM 문서로 이어질 것입니다.
NCF
2017 년에 연구원 그룹은작업신경 협업 필터링에 대해.여기에는 신경망을 사용한 협업 필터링에서 행렬 분해에 의해 모델링 된 기능적 관계를 학습하기위한 일반화 된 프레임 워크가 포함되어 있습니다.저자는 또한 고차 상호 작용을 달성하는 방법 (MF는 차수 2에 불과 함)과 두 접근 방식을 결합하는 방법을 설명했습니다.
일반적인 아이디어는 신경망이 (이론적으로) 모든 기능적 관계를 배울 수 있다는 것입니다.즉, 협업 필터링 모델이 표현하는 MF와의 관계도 신경망으로 학습 할 수 있습니다.NCF는 기본적으로 신경망을 통해 두 임베딩 간의 MF 내적 관계를 학습하기 위해 사용자와 항목 (표준 MF와 유사) 모두에 대한 간단한 임베딩 계층을 제안한 다음 간단한 다층 퍼셉트론 신경망이 뒤 따릅니다.
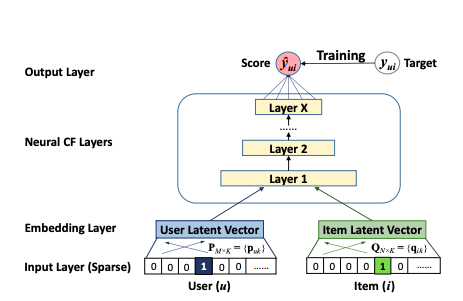
이 접근 방식의 장점은 MLP의 비선형성에 있습니다.MF에서 사용되는 간단한 내적은 항상 2 차 학습 상호 작용으로 모델을 제한하는 반면, X 레이어가있는 신경망은 이론적으로 훨씬 더 높은 수준의 상호 작용을 학습 할 수 있습니다.예를 들어 남성, 십대 및 RPG 컴퓨터 게임과 같이 상호 작용이있는 세 가지 범주 적 기능을 생각해보십시오.
실제 문제에서 우리는 임베딩에 대한 원시 입력으로 사용자 및 항목 이진화 된 벡터를 사용하는 것이 아니라 가치있을 수있는 다양한 기타 메타 또는 부가 정보 (예 : 연령, 국가, 오디오 / 텍스트 녹음, 타임 스탬프)를 분명히 포함합니다.,…) 그래서 실제로 우리는 매우 고차원적이고 매우 희소하며 연속적인 카테고리 혼합 데이터 세트를 가지고 있습니다.이 시점에서, 위에서 제시된 그림 2의 신경망은 단순한 이진 분류 피드-포워드 신경망의 형태로 콘텐츠 기반 추천으로 해석 될 수 있습니다.그리고이 해석은 CF와 콘텐츠 기반 추천 간의 하이브리드 접근 방식이되는 방식을 이해하는 데 중요합니다.네트워크는 실제로 모든 기능적 관계를 학습 할 수 있으므로 CF 차원의 3 차 이상의 상호 작용 (예 :x₁ ∙ x₂ ∙ x₃ 또는 σ (… σ (w₁x₁ + w₂x₂ + w₃x₃ + b)) 형식의 고전적인 신경망 분류 의미의 비선형 변환을 여기서 배울 수 있습니다.
고차 상호 작용을 학습하는 힘을 갖추고 있으므로 신경망을 저차 학습으로 잘 알려진 모델과 결합하여 모델이 차수 1과 2의 저차 상호 작용도 쉽게 학습 할 수 있도록 만들 수 있습니다.상호 작용, Factorization Machine.이것이 DeepFM의 저자가 논문에서 제안한 내용입니다.고차 및 저차 기능 상호 작용을 동시에 학습하기위한이 조합 아이디어는 많은 최신 추천 시스템의 핵심 부분이며 업계에서 제안 된 거의 모든 네트워크 아키텍처에서 어떤 형태로든 찾을 수 있습니다.
DeepFM
DeepFM은 FM과 심층 신경망 간의 혼합 접근 방식으로, 둘 다 동일한 입력 임베딩 레이어를 공유합니다.원시 기능은 연속 필드가 자체적으로 표현되고 범주 형 필드가 원-핫 인코딩되도록 변환됩니다.NN의 마지막 레이어에서 제공하는 최종 (예 : CTR) 예측은 다음과 같이 정의됩니다.

이것은 두 네트워크 구성 요소 인 FM 구성 요소와 Deep 구성 요소의 시그 모이 드 활성화 합계입니다.
그만큼FM 성분신경망 아키텍처 스타일로 꾸민 일반 Factorization Machine입니다.

FM 레이어의 덧셈 부분은 원시 입력 벡터 x를 직접 가져 와서 (Sparse Features Layer) 각 요소에 가중치를 곱한 다음 ( “Normal Connection”) 합산합니다.FM 레이어의 Inner Product 부분도 원시 입력 x를 가져 오지만, 임베딩 레이어를 통과 한 후에 만 임베딩 벡터 사이에 가중치 (“Weight-1 Connection”)없이 내적을 취합니다.다른 “Weight-1 연결”을 통해 두 부분을 함께 추가하면 앞서 언급 한 FM 방정식이 생성됩니다.

이 방정식에서 xᵢxⱼ 곱셈은 i = 1에서 n까지의 합을 쓸 수있을 때만 필요합니다.실제로 신경망 계산의 일부가 아닙니다.네트워크는 임베딩 레이어 아키텍처로 인해 내적을 취하기 위해 어떤 임베딩 벡터 vᵢ, vⱼ를 자동으로 인식합니다.
이 임베딩 레이어 아키텍처는 다음과 같습니다.
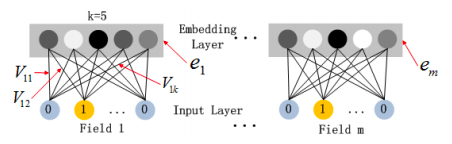
Vᵖ는 k 개의 열이있는 각 필드 p = {1,…, m}에 대한 임베딩 행렬이지만 필드의 이진화 된 버전에는 요소가 있습니다.따라서 임베딩 레이어의 출력은 다음과 같이 제공됩니다.

이것은 완전히 연결된 레이어가 아닙니다. 즉, 필드의 원시 입력과 다른 필드의 임베딩간에 연결이 없다는 점에 유의해야합니다.이렇게 생각해보십시오. 성별에 대한 원-핫 인코딩 벡터 (예 : (0,1))는 평일의 임베딩 벡터와 관련이 없습니다 (예 : (0,1,0,0,0,0,0))원시 이진화 된 평일 “화요일”이며 예를 들어 k = 4; (12,4,5,9))와 함께 벡터를 임베딩합니다.
Factorization Machine 인 FM 구성 요소는 차수 1 및 차수 2 상호 작용의 높은 중요성을 반영하며, 이는 Deep 구성 요소 출력에 직접 추가되고 최종 레이어의 시그 모이 드 활성화에 공급됩니다.
그만큼깊은 구성 요소이론상 모든 심층 신경망 아키텍처로 제안됩니다.저자는 특히 일반적인 피드 포워드 MLP 신경망 (소위 PNN)을 살펴 보았습니다.일반 MLP는 다음 그림에 나와 있습니다.
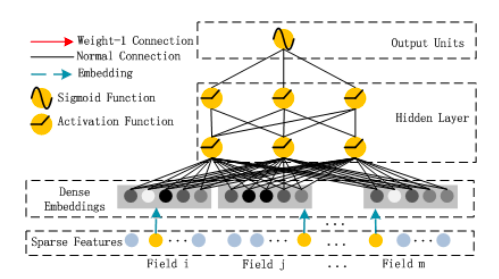
원시 데이터 (원-핫 인코딩 된 범주 입력으로 인해 매우 희소)와 다음과 같은 신경망 계층 사이에 임베딩 계층이있는 표준 MLP 네트워크 :

σ는 활성화 함수, W는 가중치 행렬, a는 이전 계층의 활성화, b는 편향입니다.
이를 통해 전반적인 DeepFM 네트워크 아키텍처가 생성됩니다.
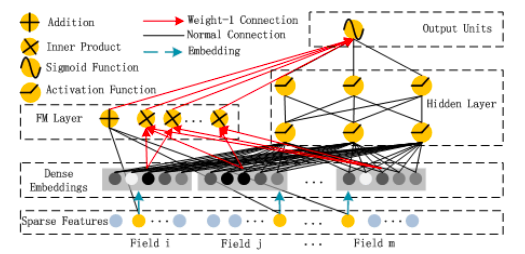
매개 변수와 함께 :
- 특성 i와 다른 특성 (임베딩 레이어) 간의 상호 작용의 영향을 측정하기위한 잠재 벡터 Vᵢ
- Vᵢ은 FM 구성 요소로 전달되어 주문 2 상호 작용 (FM 구성 요소)을 모델링합니다.
- 원시 특성 i (FM 구성 요소)의 순서 1 중요성에 가중치 부여
- Vᵢ는 또한 모든 고차 상호 작용을 모델링하기 위해 Deep 구성 요소로 전달됩니다 (& gt; 2) (Deep 구성 요소).
- Wˡ 및 bˡ, 신경망 가중치 및 편향 (Deep Component)
고차 및 저차 상호 작용을 동시에 얻는 열쇠는 특히 FM과 Deep 구성 요소 모두에 대해 동일한 임베딩 레이어를 사용하여 하나의 비용 함수로 모든 매개 변수를 동시에 훈련하는 것입니다.
Wide & amp; Deep 및 NeuMF와 비교
이 아키텍처를 잠재적으로 더 좋게 만드는 방법에 대해 상상할 수있는 많은 변형이 있습니다.그러나 핵심은 고차 및 저차 상호 작용을 동시에 모델링하는 방법에 대한 하이브리드 접근 방식에서 모두 유사합니다.DeepFM의 저자는 또한 MLP 부분을 내장 계층과 결합 된 초기 입력으로 FM 계층을 가져 오는 심층 신경망 인 소위 PNN과 교환 할 것을 제안했습니다.
NCF 논문의 저자는 또한 NeuMF ( “Neural Matrix Factorization”)라고하는 유사한 아키텍처를 내놓았습니다.FM을 하위 구성 요소로 사용하는 대신 활성화 함수에 공급되는 정규 행렬 분해를 사용했습니다.그러나이 접근 방식에는 FM의 선형 부분에 의해 모델링 된 특정 순서 1 상호 작용이 없습니다.또한 저자는 MLP 부분뿐만 아니라 행렬 분해에 대해 모델이 다른 사용자 및 항목 임베딩을 학습 할 수 있도록 특별히 허용했습니다.
앞서 언급했듯이 Google의 연구팀은 하이브리드 추천 접근 방식을위한 신경망을 최초로 제안한 팀 중 하나였습니다.DeepFM은 다음과 같은 Google의 Wide & amp; Deep 알고리즘의 추가 개발이라고 생각할 수 있습니다.
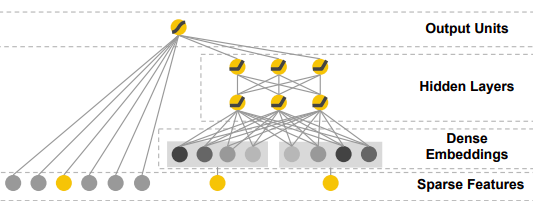
오른쪽은 임베딩 레이어가있는 잘 알려진 MLP이지만 왼쪽에는 최종 전체 출력 장치에 직접 공급되는 다른 수동 엔지니어링 입력이 있습니다.내적 연산 형태의 저차 상호 작용은 이러한 수동 엔지니어링 기능에 숨겨져 있으며 저자는 다음과 같이 여러 가지가 될 수 있다고 말합니다.
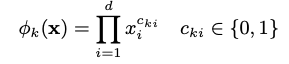
이것은 (xᵢ이 k 번째 변환의 일부인 경우 지수가 1과 같음) 서로 교차 곱하여 d 피처 (다른 이전 임베딩 포함 또는 제외) 간의 상호 작용을 캡처합니다.
DeepFM이 선험적 인 기능 엔지니어링이 필요하지 않고 하나의 공통 임베딩 레이어를 공유하는 정확히 동일한 입력 데이터에서 저차 및 고차 상호 작용을 학습 할 수 있기 때문에 얼마나 개선되었는지 쉽게 알 수 있습니다.DeepFM은 실제로 핵심 네트워크의 일부로 FM 모델을 가지고있는 반면 Wide & amp; Deep은 실제 신경망의 일부로 내적 계산을 수행하지 않고 기능 엔지니어링 단계에서 미리 수행합니다.
3. DLRM — 딥 러닝 추천 모델
따라서 Google, Huawei (DeepFM 아키텍처 관련 연구팀) 등의 다양한 옵션을 사용하여 Facebook이 사물을 보는 방식을 살펴 보겠습니다.그들은 이러한 모델의 실용적인 측면에 많은 초점을 맞춘 2019 년에 DLRM 논문을 발표했습니다.병렬 교육 설정, GPU 컴퓨팅 및 연속 기능과 범주 기능의 다양한 처리.
DLRM 아키텍처는 아래 그림에 설명되어 있으며 다음과 같이 작동합니다. 범주 형 기능은 각각 임베딩 벡터로 표시되는 반면 연속 기능은 임베딩 벡터와 동일한 길이를 갖도록 MLP에 의해 처리됩니다.이제 두 번째 단계에서는 임베딩 벡터와 처리 된 (MLP 출력) 고밀도 벡터의 모든 조합 사이의 내적이 계산됩니다.그 후, 내적은 조밀 한 특징의 MLP 출력과 연결되고 또 다른 MLP를 통과하여 마지막으로 확률을 제공하는 시그 모이 드 함수로 전달됩니다.

이 DLRM 제안은 임베딩 벡터 간의 내적 계산도 사용한다는 점에서 DeepFM의 단순화되고 수정 된 버전이지만 MLP 계층을 통해 임베딩 된 범주 형 기능을 직접 강제하지 않음으로써 고차 상호 작용에서 벗어나려고합니다..이 설계는 Factorization Machine이 임베딩 간의 2 차 상호 작용을 계산하는 방식을 모방하도록 조정되었습니다.전체 DLRM 설정을 FM 구성 요소 인 DeepFM의 특수한 부분으로 생각할 수 있습니다.DeepFM의 최종 레이어에서 FM 구성 요소의 결과에 추가 된 (그리고 시그 모이 드 함수에 공급되는) DeepFM의 고전적인 Deep Component는 DLRM 설정에서 완전히 생략 된 것으로 볼 수 있습니다.DeepFM의 이론적 이점은 설계 상 고차 상호 작용을 배우기 위해 더 잘 갖추어 졌기 때문에 분명하지만 Facebook에 따르면 다음과 같습니다.
“… 다른 네트워크에서 발견되는 2 차 이상의 고차 상호 작용은 반드시 추가 계산 / 메모리 비용의 가치가 없을 수 있습니다.”
4. 전망과 코딩
다양한 심층 추천 접근 방식, 그들의 직감, 장단점을 이론적으로 소개 한 후 제안 된 내용을 살펴 보았습니다.PyTorch 구현Facebook의 GitHub 페이지에서 DLRM의.
구현의 세부 사항을 확인하고 다양한 원시 데이터 세트를 직접 처리하기 위해 내장 된 사전 정의 된 데이터 세트 API를 사용해 보았습니다.둘 다Kaggle 디스플레이 광고 도전Criteo뿐만 아니라테라 바이트 데이터 세트사전 구현되고 다운로드 할 수 있으며 이후에 단 하나의 bash 명령으로 전체 DLRM을 훈련하는 데 사용할 수 있습니다 (지침은 DLRM repo 참조).그런 다음 Facebook의 DLRM 모델 API를 확장하여 다른 데이터 세트에 대한 전처리 및 데이터로드 단계를 포함합니다.2020 DIGIX 광고 CTR 예측.그것을 확인하시기 바랍니다여기.
digix 데이터를 다운로드하고 압축을 푼 후 비슷한 방식으로 이제 단일 bash 명령으로이 데이터에 대한 모델을 학습 할 수 있습니다.모든 전처리 단계, 임베딩의 모양 및 신경망 아키텍처 매개 변수는 digix 데이터 세트를 처리하도록 조정됩니다.명령을 안내하는 노트북을 찾을 수 있습니다.여기.digix 데이터 뒤에 숨겨진 원시 데이터와 광고 프로세스를 더 잘 이해하여 성능을 향상시키기 위해 계속 노력하고 있기 때문에 모델은 괜찮은 결과를 제공합니다.특정 데이터 정리, 하이퍼 파라미터 튜닝 및 기능 엔지니어링은 모두 제가 추가로 작업하고 싶은 작업이며공책.첫 번째 목표는 원시 digix 데이터를 입력으로 사용할 수있는 DLRM 모델 API의 기술적으로 건전한 확장을 갖는 것이 었습니다.
대체로 하이브리드 딥 모델은 추천 작업을 해결하는 가장 강력한 도구 중 하나라고 생각합니다.그러나 최근에 협업 필터링 문제를 해결하는 데있어 매우 흥미롭고 창의적인 감독되지 않은 접근 방식이 있습니다.오토 인코더.따라서이 시점에서 저는 오늘날 거대 인터넷 거대 기업이 우리가 클릭 할 가능성이 가장 높은 광고를 제공하기 위해 무엇을 사용하고 있는지 추측 할 수 있습니다.앞서 언급 한 오토 인코더 접근 방식과이 기사에 제시된 딥 하이브리드 모델의 일부 형태의 조합이 될 수 있다고 가정합니다.
참고 문헌
스테 펜 렌들.분해 기계.Proc.데이터 마이닝에 관한 2010 IEEE 국제 컨퍼런스, 페이지 995–1000, 2010.
Xiangnan He, Lizi Liao, Hanwang Zhang, Liqiang Nie, Xia Hu 및 Tat-Seng Chua.신경 협업 필터링.Proc.26th Int.Conf.World Wide Web, 페이지 173–182, 2017.
Huifeng Guo, Ruiming Tang, Yunming Ye, Zhenguo Li, Xiuqiang He.DeepFM : CTR 예측을위한 분해 기계 기반 신경망.arXiv 사전 인쇄 arXiv : 1703.04247, 2017.
Jianxun Lian, Xiaohuan Zhou, Fuzheng Zhang, Zhongxia Chen, Xing Xie 및 Guangzhong Sun.xDeepFM : 추천 시스템에 대한 명시 적 및 암시 적 기능 상호 작용 결합.Proc.제 24 회 ACM SIGKDD International Conference on Knowledge Discovery & amp;데이터 마이닝, 1754–1763 페이지.ACM, 2018 년.
Heng-Tze Cheng, Levent Koc, Jeremiah Harmsen, Tal Shaked, Tushar Chandra, Hrishi Aradhye, Glen Anderson, Greg Corrado, Wei Chai, Mustafa Ispir, Rohan Anil, Zakaria Haque, Lichan Hong, Vihan Jain, Xiaobing Liu 및 Hemal Shah.와이드 & amp;추천 시스템을위한 딥 러닝.Proc.추천 시스템을위한 딥 러닝에 대한 1 차 워크숍, 7-10 페이지, 2016.
M. Naumov, D. Mudigere, HM Shi, J. Huang, N. Sundaraman, J. Park, X. Wang, U. Gupta, C. Wu, AG Azzolini, D. Dzhulgakov, A. Mallevich, I. Cherniavskii,Y. Lu, R. Krishnamoorthi, A. Yu, V. Kondratenko, S. Pereira, X. Chen, W. Chen, V. Rao, B. Jia, L. Xiong, M. Smelyanskiy,“딥 러닝 추천 모델개인화 및 추천 시스템,”CoRR, vol.abs / 1906.00091, 2019. [온라인].유효한:http://arxiv.org/abs/1906.00091 [39]
Modern Recommender Systems
Modern Recommender Systems
A Deep Dive into the AI algorithms that companies like Facebook and Google have built their business around.
As recently as May 2019 Facebook open-sourced some of their recommendation approaches and introduced the DLRM (Deep-learning Recommendation Model). This blog post is meant to explain how and why DLRM and other modern recommendation approaches work so well by looking at how they can be derived from previous results in the domain and by explaining their inner workings and intuitions in detail.
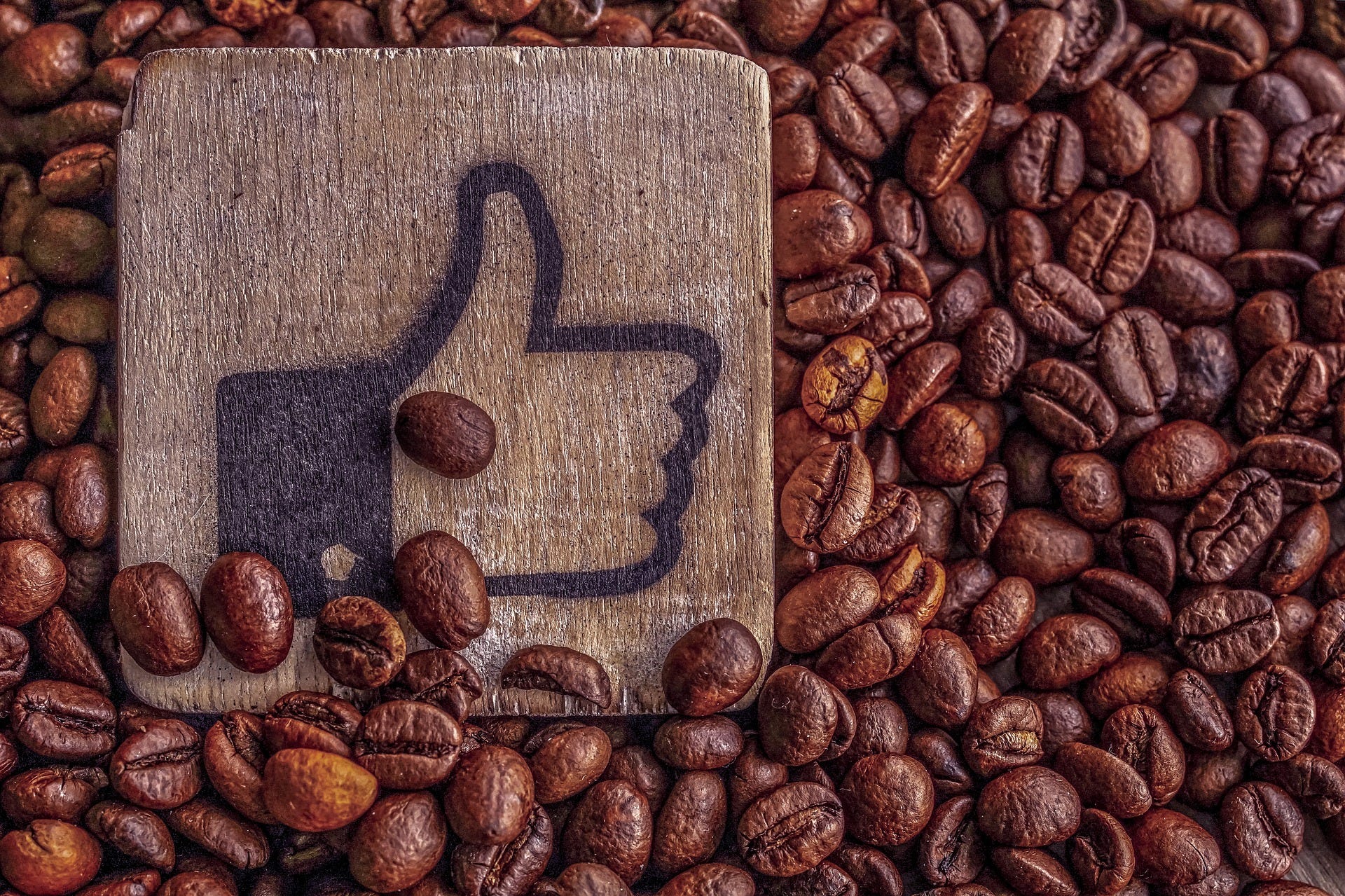
Personalized AI-based advertisement is the name of the game in online marketing these days and companies like Facebook, Google, Amazon, Netflix, and co are kings of the online marketing jungle because they have not only adopted this trend but have essentially invented it and built their entire business strategies around it. Netflix’s “other movies you might enjoy” or Amazon’s “Customers who bought this item also bought…” are just some examples of many in the online world.
So naturally, the everyday Facebook and Gooogle user that I am, I asked myself at some point:
“HOW EXACTLY DOES THIS THING WORK?“
And yeah, we all know the basic movie recommendation example to explain how collaborative filtering/matrix factorization works. Also, I am not talking about the approach of training a straight-forward classifier per user, that outputs a probability of whether or not that user likes a certain product. Those two approaches, namely collaborative filtering and content-based recommendation have to yield some sort of performance and some predictions that can be used, but Google, Facebook, and co surely must have something better up their sleeves, otherwise they wouldn’t be where they are today.
In order to understand where today’s high-end recommendation systems come from, we have to take a look at two of the basic approaches to the problem of
predicting how much a certain user likes a certain item.
which in the online-marketing world adds up to predicting click-through rates (CTR) for possible ads, based on explicit feedback such as ratings, likes, etc. as well as implicit feedback, such as clicks, search histories, comments, or website visits.
Content-based filtering vs. Collaborative-filtering
1. Content-based filtering
Loosely speaking content-based recommendation means to predict whether a user likes a certain product by using the user’s online history. That includes, among others, likes the user gave (e.g. on Facebook), keywords he/she searched for (e.g. on Google), and simply clicks and visits he/she made to certain websites. All in all, it focuses on the user’s own preferences. We can for example think of a simple binary classifier (or regressor) that outputs a click-through rate (or rating) for a certain ad-group for this user.
2. Collaborative-filtering
Collaborative filtering however tries to predict whether a user might like a certain product by looking at the preferences of similar users. Here we can think of the standard matrix factorization (MF) approach for movie recommendations where the ratings matrix get’s factorized into one embedding matrix for the users and one for the movies.
A disadvantage of classic MF is that we cannot use any side features e.g. movie genre, release date, etc., the MF itself has to learn them from the existing interactions. Also, MF suffers from the so-called “cold start problem”, meaning a new movie that hasn’t been rated by anyone yet, cannot be recommended. Content-based filtering solves these two issues, however, is lacking the predictive power of looking at similar users’ preferences.
The advantages and disadvantages of the two different approaches bring up very clearly the need for a hybrid approach where both ideas are somehow combined into one model.
Hybrid recommendation models
1. Factorization Machine
One idea that was introduced by Steffen Rendle in 2010 is the Factorization Machine. It holds the basic mathematical approach to combining matrix factorization with regression
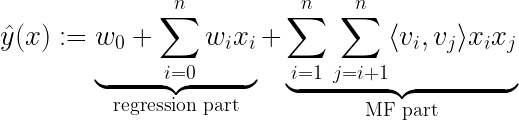
where the model parameters that need to be estimated during learning are:

and ⟨ ∙ , ∙ ⟩ is the dot product between two vectors vᵢ and vⱼof size ℝᵏ, who can be seen as rows in V.
It is pretty straight-forward to see how this equation makes sense when looking at an example of how to represent the data x that gets thrown into this model. Let’s have a look at the described example in the paper on Factorization Machines by Steffen Rendle:
Imagine having the following transaction data on movie reviews where users give ratings to movies at a certain time:
- user u ∈ U = {Alice (A), Bob (B), . . .}
- movie (item) i ∈ I = {Titanic (TI), Notting Hill (NH), Star Wars (SW), Star Trek (ST), . . .}
- rating r ∈ {1,2,3,4,5} at time t ∈ ℝ

Looking at the figure above we can see the data setup for a hybrid recommendation model. Both the sparse features that represent the user and the item as well as any additional meta or side information (e.g. “Time” or “Last Movie Rated” in this example) are part of a feature vector x that gets mapped to a target y. Now the key is how they are processed by the model.
- The regression part of the FM handles both the sparse data (e.g. “User”) as well as the dense data (e.g. “Time”) like a standard regression task and thus can be interpreted as the content-based filtering approach within the FM.
- The MF part of the FM now accounts for the interactions between feature blocks (e.g. interaction between “User” and “Movie”), where the matrix V can be interpreted as the embedding matrix used in collaborative filtering approaches. These cross-user-movie relationships, bring us insights such as:
user i who has a similar embedding vᵢ (representing his preferences for movie attributes) as another user j with embedding vⱼ, might very well like similar movies as user j.
Adding the two predictions of the regression part and the MF part together and learning their parameters simultaneously in one cost function leads to the hybrid FM model that now uses a “best of both worlds” approach to making a recommendation for a user.
This hybrid approach of a Factorization Machine at first glance already seems to be a perfect “best of both worlds” model, however, as many different AI fields like NLP or computer vision have proven in the past:
“Throw it in a Neural Net and you will make it even better”
2. Wide and Deep, Neural Collaborative Filtering (NCF) and Deep Factorization Machines (DeepFM)
We will first have a look at how collaborative filtering can be solved by a neural net approach by looking at the NCF paper, this will lead us to Deep Factorization Machines (DeepFM) which are a neural net version of factorization machines. We will see why they are superior to regular FMs and how we can interpret the neural net architecture. We will see how DeepFM was developed as an improvement to the previously released Wide&Deep model by Google, which is one of the first major breakthroughs of deep learning in recommendation systems. This will finally lead us to the aforementioned DLRM paper, released by Facebook in 2019, that can be seen as a simplification and slight adjustment to DeepFM.
NCF
In 2017 a group of researchers released their work on Neural Collaborative Filtering. It contains a generalized framework for learning the functional relationship modeled by matrix factorization in collaborative filtering with a neural network. The authors also explained how to achieve higher-order interactions (MF is only order 2) and how to fuse the two approaches together.
The general idea is that a neural network can (in theory) learn any functional relationship. That means that also the relationship a collaborative filtering model expresses with it’s MF can be learned by a neural net. NCF proposes a simple embedding layer for both users and items (similar to standard MF) followed by a straight-forward multi-layer perceptron neural net to basically learn the MF dot product relationship between the two embeddings via neural net.
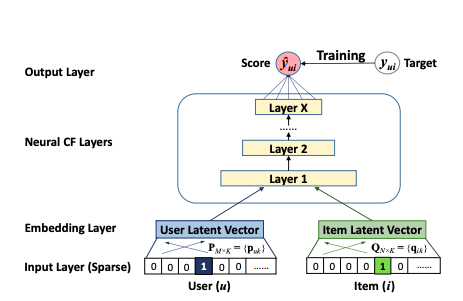
The advantage of this approach lies in the non-linearity of the MLP. The simple dot product used in MF will always limit the model to learning interactions of degree 2, whereas a neural net with X layers can in theory learn interactions of a much higher degree. Think of 3 categorical features that all have an interaction, like male, teenager, and RPG computer games for example.
In real-world problems, we don’t just use a user and an item binarized vector as raw input to our embeddings but obviously include various other meta or side information that might be valuable (e.g. age, country, audio/text recordings, timestamp, …) so in reality we have a very high-dimensional, highly sparse and continuous-categorical mixed dataset. At this point, the above presented neural net from Fig. 2 could very well also be interpreted as a content-based recommendation in the form of a simple binary classification feed-forward neural net. And this interpretation is key to understanding how it ends up being a hybrid approach between CF and content-based recommendation. The network can in fact learn any functional relationship, thus interactions in the CF sense of degree 3 or higher, e.g. x₁ ∙ x₂ ∙ x₃, or any non-linear transformation in the classical neural net classification sense of the form σ( … σ(w₁x₁+w₂x₂ + w₃x₃ + b)) can be learned here.
Equipped with the power of learning high-order interactions, we can specifically make it easy for our model to learn also the low order interactions of order 1 and 2, by combining the neural net with a model that is well known to learn low-order interactions, the Factorization Machine. That’s exactly what the authors of DeepFM proposed in their paper. This combination idea, to simultaneously learn high and low-order feature interactions, is the key part of many modern recommender systems and can be found in some form or another in almost every network architecture proposed in the industry.
DeepFM
DeepFM is a mixed approach between FM and a deep neural network, that both share the same input embedding layer. Raw features are transformed such that continuous fields are represented by themselves and categorical fields are one-hot encoded. The final (e.g. CTR) prediction, given by the last layer in the NN is defined as:

which is a sigmoid activated sum of the two network components: the FM component and the Deep component.
The FM component is a regular Factorization Machine dressed up in neural net architecture style:

The Addition part of the FM Layer gets the raw input vector x directly (Sparse Features Layer) and multiplies each element with its weight (“Normal Connection”) before summing them up. The Inner Product part of the FM Layer also gets the raw inputs x, but only after they have been passed through the embedding layer and simply takes the dot product without any weight (“Weight-1 Connection”) between the embedding vectors. Adding the two parts together through another “Weight-1 Connection” yields the aforementioned FM equation:

The xᵢxⱼ multiplication in this equation is only needed to be able to write the sum over i=1 through n. It isn’t really part of the neural network computation. The network automatically knows which embedding vectors vᵢ, vⱼ to take the dot product between due to the embedding layer architecture.
This embedding layer architecture looks as follows:
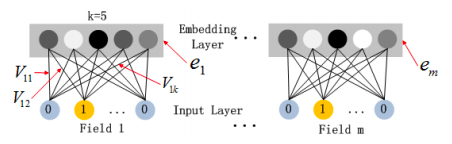
with Vᵖ being the embedding matrix for each field p={1,…,m} with k columns and however many rows the binarized version of the field has elements. The output of the embedding layer is thus given as:

and it is important to note that this is not a fully connected layer, namely there is no connection between any field’s raw inputs and any other field’s embedding. Think of it this way: the one-hot encoded vector for gender (e.g. (0,1)) cannot have anything to do with the embedding vector for weekday (e.g. (0,1,0,0,0,0,0) raw binarized weekday “Tuesday” and it’s embedding vector with e.g.: k=4; (12,4,5,9)).
The FM component being a Factorization Machine reflects the high importance of both order 1 and order 2 interactions, which are directly added to the Deep component output and fed into the sigmoid activation in the final layer.
The Deep Component is proposed to be any deep neural net architecture in theory. The authors specifically took a look at a regular feed-forward MLP neural net (as well as a so-called PNN). The regular MLP is given by the following figure:
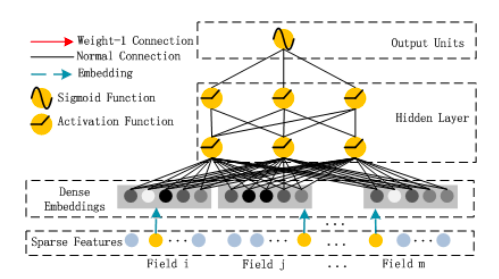
a standard MLP network with embedding layer between the raw data (highly sparse due to one-hot-encoded categorical input) and the following neural net layers given as:

with σ the activation function, W the weight matrix, a the activation from the previous layer, and b the bias.
This yields the overall DeepFM network architecture:
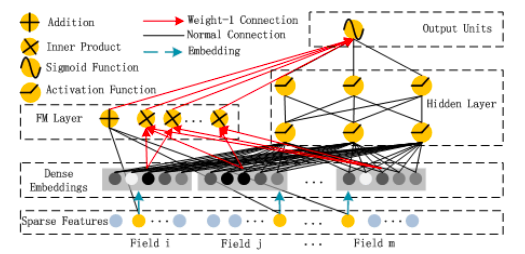
with the parameters:
- latent vector Vᵢ to measure impact of feature i’s interactions with other features (Embedding layer)
- Vᵢ gets passed to the FM component to model order-2 interactions (FM Component)
- wᵢ weighting the order 1 importance of raw feature i (FM Component)
- Vᵢ also gets passed to the Deep component to model all higher-order interactions (>2) (Deep Component)
- Wˡ and bˡ, the neural net’s weights and biasses (Deep Component)
The key to getting both high and low order interactions simultaneously is training all parameters at the same time under one cost function, specifically using the same embedding layer for both the FM as well as the Deep component.
Comparison to Wide&Deep and NeuMF
There are many variations that one can dream up on how to tweak this architecture to potentially make it even better. At the core, however, they are all similar in their hybrid approach on how to model high and low order interactions simultaneously. The authors of DeepFM also proposed interchanging the MLP part with a so-called PNN, a deep neural network that gets the FM layer as initial input combined with the embedding layer
The authors of the NCF paper also came up with a similar architecture which they called NeuMF (“Neural Matrix Factorization”). Instead of having an FM as low-order component, they used a regular matrix factorization fed into an activation function. This approach however is lacking the specific order 1 interactions modeled by the linear part of the FM. Also, the authors specifically allowed the model to learn different user and item embeddings for the matrix factorization as well as the MLP part.
As mentioned before, Google’s research team was one of the first to propose a neural network for a hybrid recommendation approach. DeepFM can be thought of as a further development of Google’s Wide&Deep algorithm that looks like this:
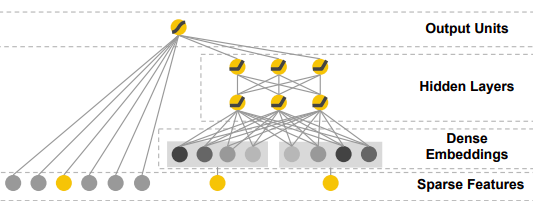
The right side is our well-known MLP with an embedding layer, the left side however has different, manually engineered, inputs that are directly fed into the final overall output unit. The low-order interaction in the form of the dot product operation is hidden in these manually engineered features, that the authors say can be many different things, for example:
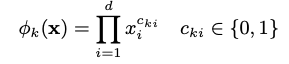
which captures the interactions between d features (with or without another previous embedding) by cross multiplying them (exponent equals 1 if xᵢ is part of k-th transformation) with each other.
It is easy to see how DeepFM is an improvement since it does not require any a priori feature engineering and is able to learn low and high-order interactions from exactly the same input data that all share one common embedding layer. DeepFM really has the FM model as part of its core network, whereas Wide&Deep does not do dot product computations as part of the actual neural net but beforehand in feature engineering steps.
3. DLRM — Deep Learning Recommendation Model
So with all these different options from Google, Huawei (research team around the DeepFM architecture), and others, let’s take a look at how Facebook views things. They came out with their DLRM paper in 2019, which focuses a lot on the practical side of these models. Parallel training setup, GPU computing as well as different handling of continuous vs categorical features.
The DLRM architecture is described in the below figure and works as follows: Categorical features are each represented by an embedding vector, while continuous features are processed by an MLP such that they have the same length as the embedding vectors. Now in a second stage, the dot product between all combinations of embedding vectors and processed (MLP output) dense vectors is computed. Afterward, the dot products are concatenated with the MLP output of the dense features and passed through another MLP and finally into a sigmoid function to give a probability.

This DLRM proposal is somewhat of a simplified and modified version of DeepFM in the sense that it also uses dot product computations between embedding vectors but it specifically tries to stay away from high-order interactions by not directly forcing the embedded categorical features through an MLP layer. The design is tailored to mimic the way Factorization Machines compute the second-order interactions between the embeddings. We can think of the entire DLRM setup as the specialized part of DeepFM, the FM component. The classical Deep Component of DeepFM that gets added to the outcome of the FM component in the final layer of DeepFM (and then fed into a sigmoid function) can be seen as completely omitted in the DLRM setup. The theoretical advantages of DeepFM are clear as it is, by design, better equipped to learn high order interaction, however according to Facebook:
“… higherorder interactions beyond second-order found in other networks may not necessarily be worth the additional computational/memory cost”
4. Outlook and Coding
Having introduced various deep recommendation approaches, their intuitions as well as pros and cons, in theory, I had a look at the proposed PyTorch implementation of DLRM on Facebook’s GitHub page.
I checked out the details of the implementation and tried out the predefined dataset APIs that they have built-in to handle different raw datasets directly. Both the Kaggle display advertising challenge by Criteo as well as their Terabyte dataset are pre implemented and can be downloaded and subsequently used to train a full DLRM with just one bash command (see DLRM repo for instructions). I then extended Facebook’s DLRM model API to include preprocessing and data loading steps for another dataset, the 2020 DIGIX Advertisement CTR Prediction. Please check it out here.
In a similar fashion after downloading and unzipping the digix data you can now train a model on this data with a single bash command as well. All the preprocessing steps, shapes of the embeddings, and neural net architecture parameters are adjusted towards handling the digix dataset. A notebook that takes you through the commands can be found here. The model delivers some decent results, as I am continuing to work on it to improve the performance by understanding the raw data and the advertisement process behind the digix data better. Specific data cleaning, hyperparameter tuning, and feature engineering are all things I would like to further work on and are mentioned in the notebook. The first goal was simply to have a technically sound extension of the DLRM model API that can use the raw digix data as input.
All in all, I believe hybrid deep models are one of the most powerful tools for solving recommendation tasks. However, there have been some seriously interesting and creative unsupervised approaches on solving collaborative filtering problems recently using autoencoders. So at this point, I can only guess what the large internet giants are using today to feed us ads we are most likely to click on. I assume that it very well could be a combo of the aforementioned autoencoder approach as well as some form of the deep hybrid models presented in this article.
References
Steffen Rendle. Factorization machines. In Proc. 2010 IEEE International Conference on Data Mining, pages 995–1000, 2010.
Xiangnan He, Lizi Liao, Hanwang Zhang, Liqiang Nie, Xia Hu, and Tat-Seng Chua. Neural collaborative filtering. In Proc. 26th Int. Conf. World Wide Web, pages 173–182, 2017.
Huifeng Guo, Ruiming Tang, Yunming Ye, Zhenguo Li, and Xiuqiang He. DeepFM: a factorizationmachine based neural network for CTR prediction. arXiv preprint arXiv:1703.04247, 2017.
Jianxun Lian, Xiaohuan Zhou, Fuzheng Zhang, Zhongxia Chen, Xing Xie, and Guangzhong Sun. xDeepFM: Combining explicit and implicit feature interactions for recommender systems. In Proc. of the 24th ACM SIGKDD International Conference on Knowledge Discovery & Data Mining, pages 1754–1763. ACM, 2018.
Heng-Tze Cheng, Levent Koc, Jeremiah Harmsen, Tal Shaked, Tushar Chandra, Hrishi Aradhye, Glen Anderson, Greg Corrado, Wei Chai, Mustafa Ispir, Rohan Anil, Zakaria Haque, Lichan Hong, Vihan Jain, Xiaobing Liu, and Hemal Shah. Wide & deep learning for recommender systems. In Proc. 1st Workshop on Deep Learning for Recommender Systems, pages 7–10, 2016.
M. Naumov, D. Mudigere, H. M. Shi, J. Huang, N. Sundaraman, J. Park, X. Wang, U. Gupta, C. Wu, A. G. Azzolini, D. Dzhulgakov, A. Mallevich, I. Cherniavskii, Y. Lu, R. Krishnamoorthi, A. Yu, V. Kondratenko, S. Pereira, X. Chen, W. Chen, V. Rao, B. Jia, L. Xiong, and M. Smelyanskiy, “Deep learning recommendation model for personalization and recommendation systems,” CoRR, vol. abs/1906.00091, 2019. [Online]. Available: http://arxiv.org/abs/1906. 00091 [39]