The Three Decoding Methods For NLP
From greedy to beam search
One of the often-overlooked parts of sequence generation in natural language processing (NLP) is how we select our output tokens — otherwise known as decoding.
You may be thinking — we select a token/word/character based on the probability of each token assigned by our model.
This is half-true — in language-based tasks, we typically build a model which outputs a set of probabilities to an array where each value in that array represents the probability of a specific word/token.
At this point, it might seem logical to select the token with the highest probability? Well, not really — this can create some unforeseen consequences — as we will see soon.
When we are selecting a token in machine-generated text, we have a few alternative methods for performing this decode — and options for modifying the exact behavior too.
In this article we will explore three different methods for selecting our output token, these are:
> Greedy Decoding> Random Sampling> Beam Search
It’s pretty important to understand how each of these works — often-times in language applications, the solution to a poor output can be a simple switch between these four methods.
If you’d prefer video, I cover all three methods here too:
I’ve included a notebook for testing each of these methods with GPT-2 here.
Greedy Decoding
The simplest option we have is greedy decoding. This takes our list of potential outputs and the probability distribution already calculated — and chooses the option with the highest probability (argmax).
It would seem that this is entirely logical — and in many cases, it works perfectly well. However, for longer sequences, this can cause some problems.
If you have ever seen an output like this:
This is most likely due to the greedy decoding method getting stuck on a particular word or sentence and repetitively assigning these sets of words the highest probability again and again.
Random Sampling
The next option we have is random sampling. Much like before we have our potential outputs and their probability distribution.
Random sampling chooses the next word based on these probabilities — so in our example, we might have the following words and probability distribution:
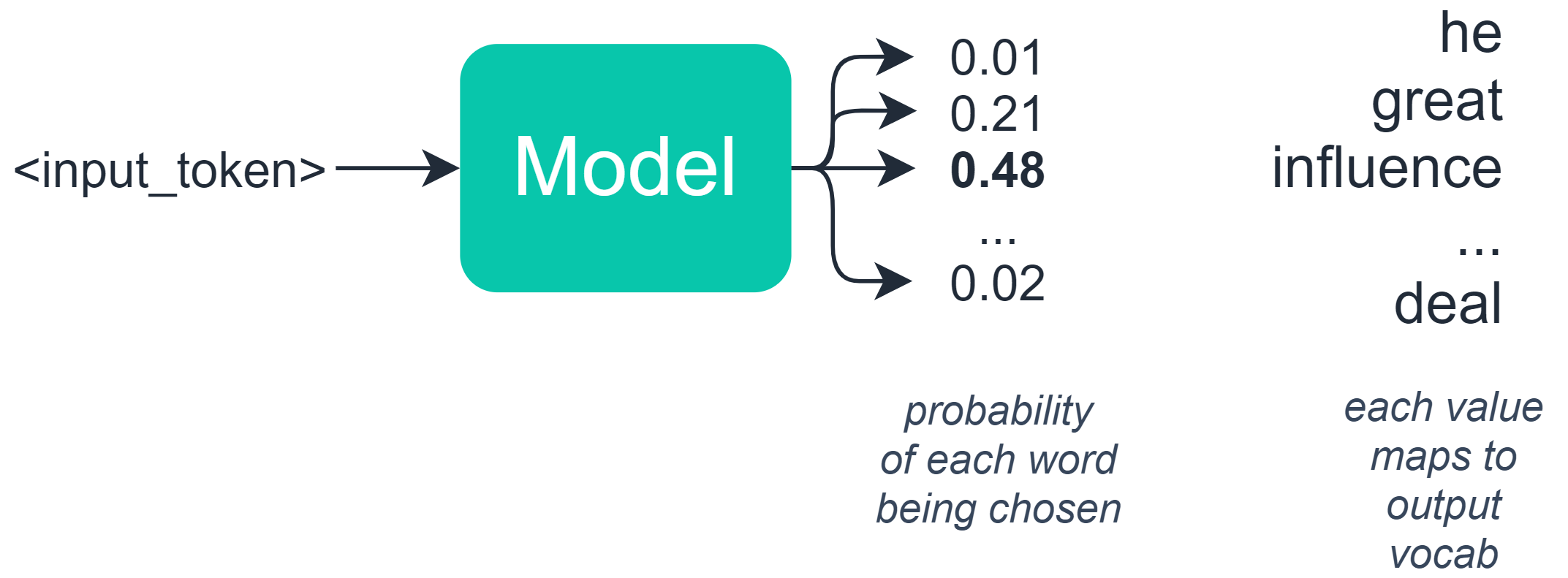
We would have a 48% chance of the model selecting ‘influence’, a 21% chance of it choosing ‘great’, and so on.
This solves our problem of getting stuck in a repeating loop of the same words because we add randomness to our predictions.
However, this introduces a different problem — we will often find that this approach can be too random and lacks coherence:
So on one side, we have greedy search which is too strict for generating text — on the other we have random sampling which produces wonderful gibberish.
We need to find something that does a little of both.
Beam Search
Beam search allows us to explore multiple levels of our output before selecting the best option.
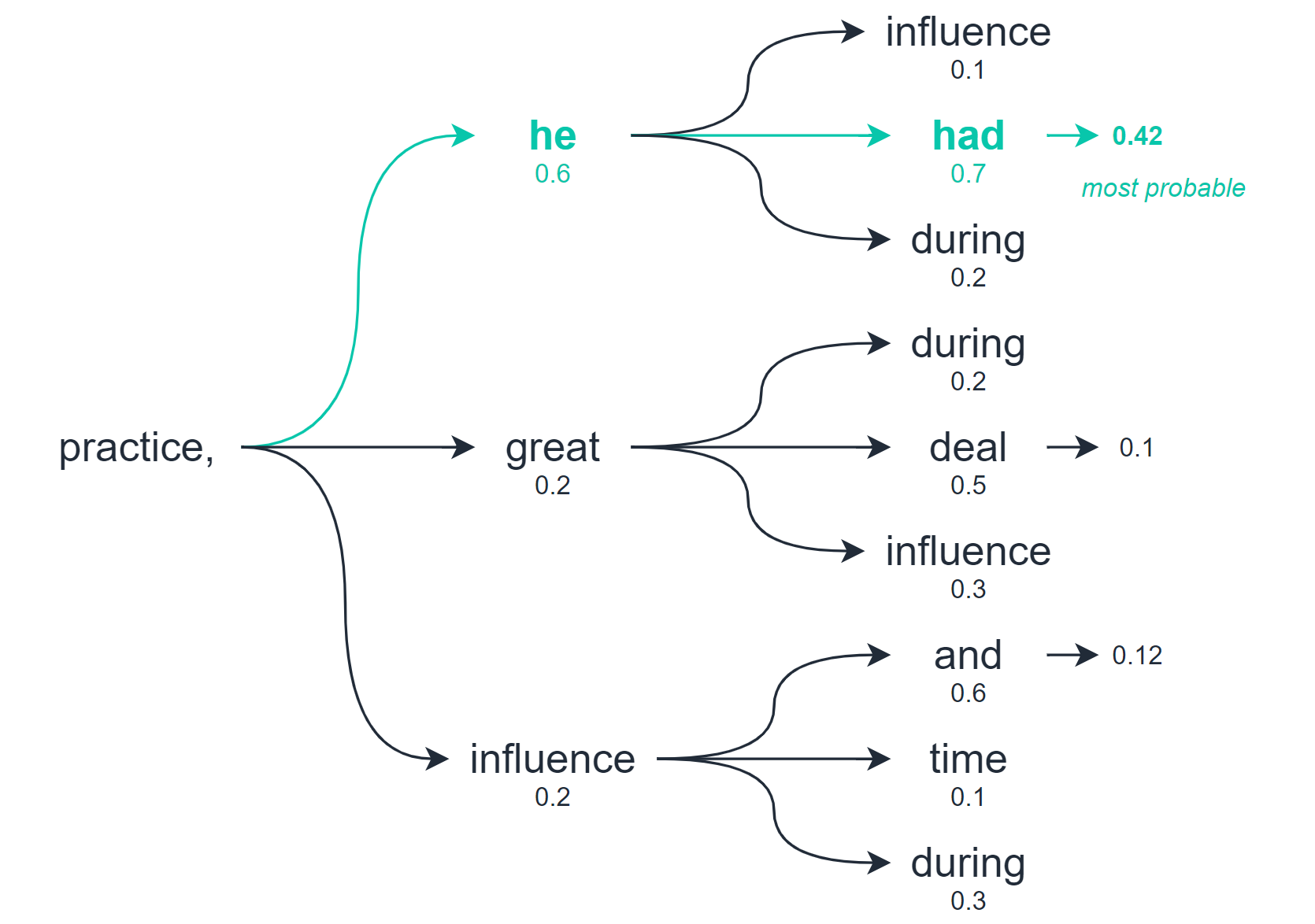
So whereas greedy decoding and random sampling calculate the best option based on the very next word/token only — beam search checks for multiple word/tokens into the future and assesses the quality of all of these tokens combined.
From this search, we will return multiple potential output sequences — the number of options we consider is the number of ‘beams’ we search with.
However, because we are now back to ranking sequences and selecting the most probable — beam search can cause our text generation to again degrade into repetitive sequences:
So, to counteract this, we increase the decoding temperature
— which controls the amount of randomness in the outputs. The default temperature
is 1.0
— pushing this to a slightly higher value of 1.2
makes a huge difference:
With these modifications, we have a peculiar but nonetheless coherent output.
That’s it for this summary of text generation decoding methods!
I hope you’ve enjoyed the article, let me know if you have any questions or suggestions via Twitter or in the comments below.
Thanks for reading!
If you’re interested in learning how to set up a text generation model in Python, I wrote this short article covering how here:
*All images are by the author except where stated otherwise